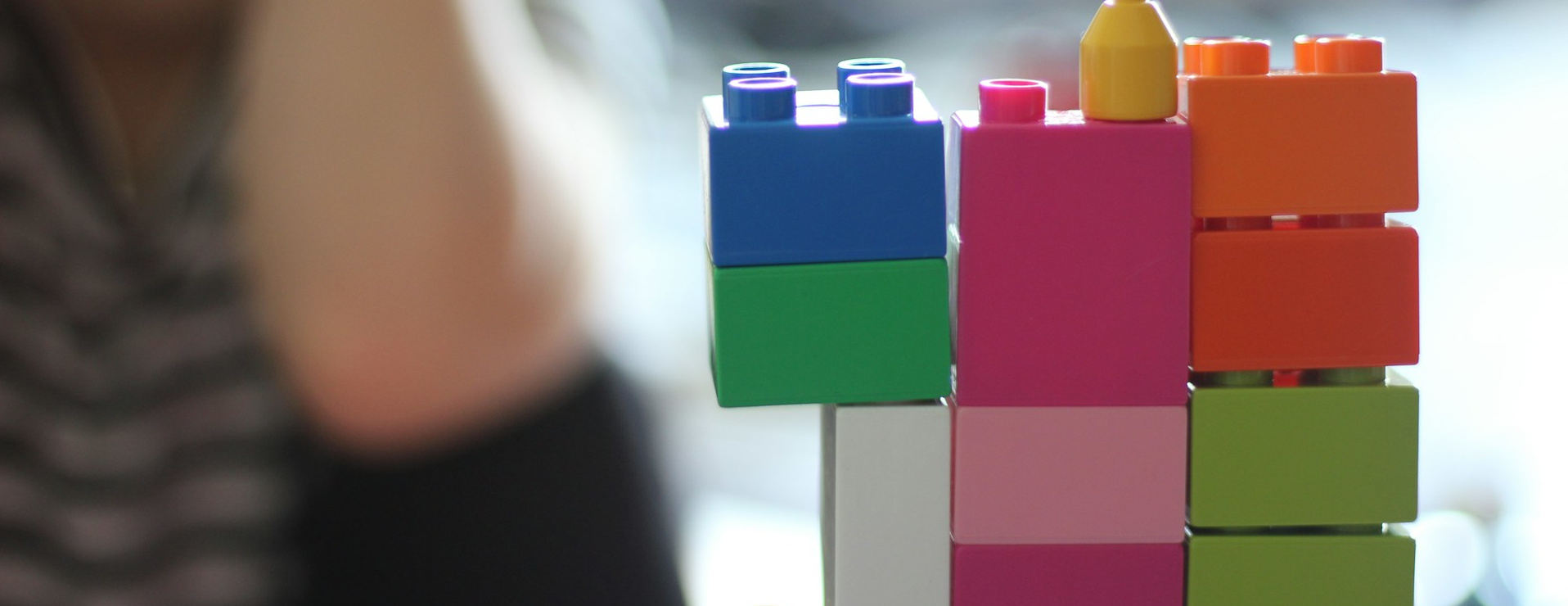
Starting words
Hello again! We are continuing our series on extracting Machine Learning training data from a video game. In the previous blog entry, we’ve run some exploratory data analysis on what we aim to extract for the "final" model’s training. From now on, we’ll be focusing on the actual extraction process of said data.
The theme of this particular post is "ready-made". In other words, we’re going to look at some relatively current methods to solve our problem – or side step it; ones that have a characteristic of doing most of the work for us. We’ll start with a modern detection model, and then proceed with a local LLM (or rather, VLM), explore alternative sources of auto-derived detection training data, and compare all that with hosted LLMs from leading vendors.
The current entry is likely to be the least "technically complex" in the entire series, meaning it will be the easiest to replicate for an arbitrary person, and therefore adapt to other use cases. Something to keep in mind, especially if you, Dear Reader, stumbled upon this post randomly. |
For a refresher on what we want to achieve, feel free to consult the starter blog entry, here.
Modern Detectiving with Open-Vocabulary Models
Intro
"Modern" is, of course, an ill-defined term in the current breakneck-paced bazaar of ML solutions. However, we can provide a sensible generalization of what that could be at the moment of writing of this entry, at least for our specific problem scope.
Speaking of specific, one relatively recent – i.e., no more than 2-year-old – trend for detection models is to enable operating on an "open vocabulary", as opposed to a rigid set of classes. So, instead of telling the model to detect classes of objects like "car", "bicycle", and similar, the user can be more creative, and supply prompts such as "yellow vehicle", or "car with two windows".
As already mentioned, open-vocabulary detection models have been developed for about 2 years now. A relatively fresh example, and one we’ll use in the current section, is YOLO-World, released early 2024. True to its name, it is based on YOLO for detection, augmented by the ability to fuse text and image embeddings (representations of both in a numeric vector space). A detailed explanation is beyond the scope of this blog – for those interested, the original paper is available on arXiv.
We’ll now see if we can wrangle YOLO-World to detect our designators. To juggle our memory from the earlier entries, the designator is depicted in the center of the screenshot below, surrounding the targeted mech:
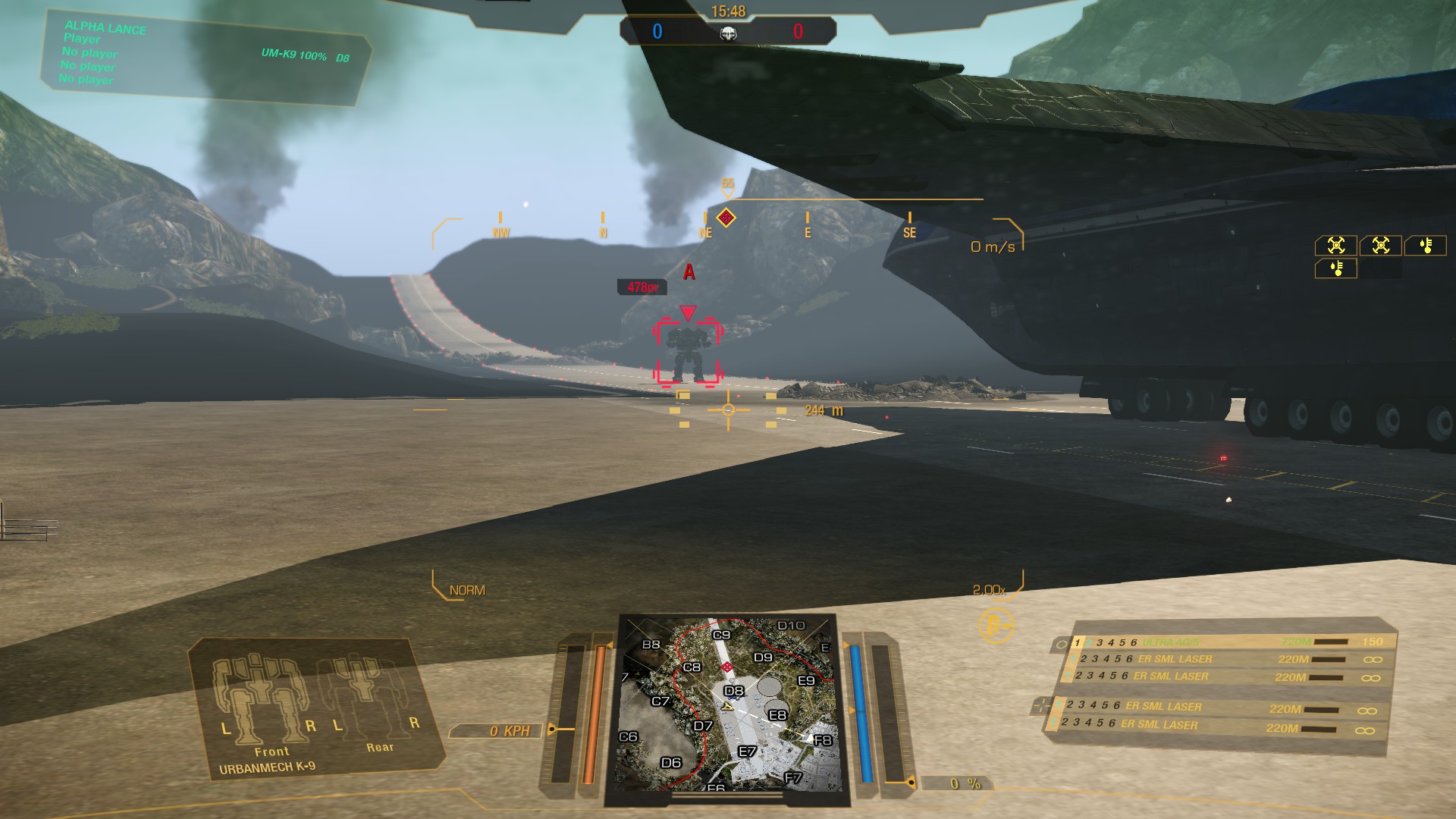
Implementation
The nice thing about testing out ML models in recent years is that, for the vast majority of use cases, everything is so, so convenient[1]. Not only are there repositories that standardize model usage and deployment – most prominent Hugging Face, but they often come "free" with libraries further expediting the use of models present within their ecosystem. On top of that, utility libraries exist that aggregate those "commonized" APIs for model usage, evaluation, and similar tasks into a meta-API, so that an arbitrary model authors' choice of standards is not as much of a pain point as it was, say, 5 years ago (yes, yes, feel free to add your favorite joke on standards proliferation here).
A library/framework that possesses the quality praised in the preceding paragraph, and one that we’ll be using here, is Supervision.
So let’s see how that goes; by "that" we mean using YOLO-World to find the target designator in the image. We’ll essentially be following along one of Supervision’s tutorials, with some small modifications:
import cv2 as cv
import supervision as sv
from supervision import Position
from inference.models.yolo_world.yolo_world import YOLOWorld
detection_model = YOLOWorld(model_id="yolo_world/l")
# several different class prompts, in the hope that at least
# one will match for the designator
classes = ["red rectangle", "red outline", "red box", "red designator"]
detection_model.set_classes(classes)
# setting up the annotators
BOUNDING_BOX_ANNOTATOR = sv.BoundingBoxAnnotator(thickness=2)
LABEL_ANNOTATOR = sv.LabelAnnotator(text_thickness=1, text_scale=0.5, text_color=sv.Color.WHITE, text_position=Position.BOTTOM_LEFT)
# loading the image shown above
frame = cv.imread("example_designator.jpg")
# we're using a very low confidence threshold, as we're
# interested in seeing "what sticks"
results = detection_model.infer(frame, confidence=1e-3)
# however, we are also still applying NMS, as potentially, in low
# confidence scenarios, we run the risk of being inundated with multiple,
# redundant, tiny detections
detections = sv.Detections.from_inference(results).with_nms(threshold=1e-4)
print(detections)
# will print out something like:
# Detections(xyxy=array([[ 709.02, 829.93, 810.31, 1055.4],
# [ 810.56, 343.53, 879.66, 390.26],
# [ 799.74, 807.5, 1123.7, 1063.1],
# [ 809.68, 343.99, 879.36, 390.05]]),
# mask=None,
# confidence=array([ 0.0019695, 0.0014907, 0.0012708, 0.0012423]),
# class_id=array([2, 2, 2, 0]),
# tracker_id=None,
# data={'class_name': array(['red box', 'red box', 'red box', 'red rectangle'], dtype='<U13')})
# this is, again, pretty much copied from the linked tutorial
# BTW, it is a bit unusual there's no sensible
# way to compose Supervision annotators
annotated_image = frame.copy()
labels = [
f"{classes[class_id]} {confidence:0.5f}"
for class_id, confidence
in zip(detections.class_id, detections.confidence)
]
annotated_image = BOUNDING_BOX_ANNOTATOR.annotate(annotated_image, detections)
annotated_image = LABEL_ANNOTATOR.annotate(annotated_image, detections, labels=labels)
sv.plot_image(annotated_image, (30, 30))
The final line results in the following image:
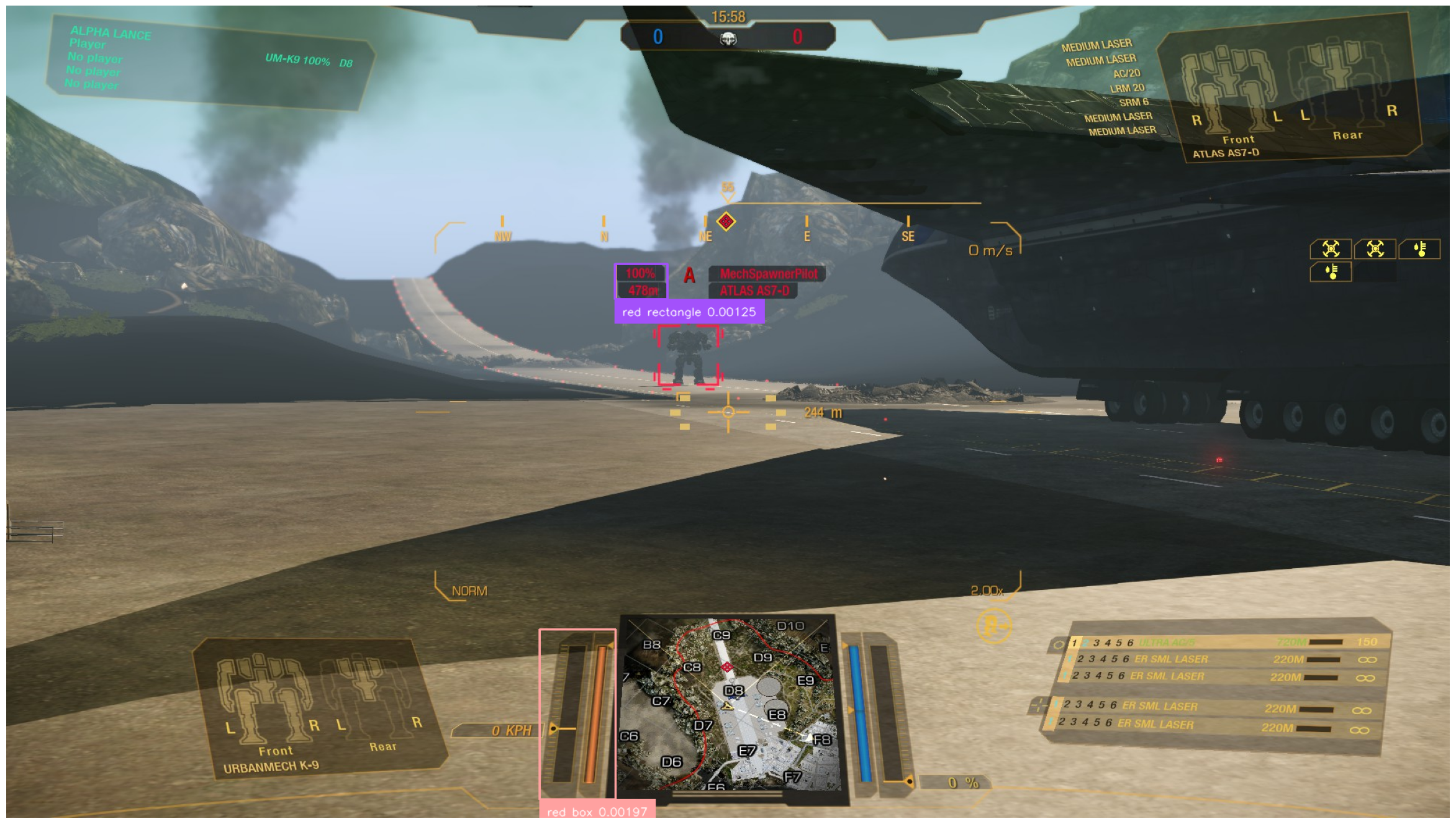
Well, that’s not looking great. The model, although powerful, evidently has trouble distinguishing various elements in our screenshot. The likely underlying reason is simple — the model was trained on datasets of "actual" images, i.e., photographs, and not on game screenshots[2].
Instead of looking at multiple (pre-)training datasets, we’ll check out the one used for evaluation, specifically LVIS. Let’s take a small look at that dataset to see what kind of text input data it provides. The spec is here – we’re interested in the "Data Format" → "Categories" section in particular. Let’s also load up the training set and see what some example data looks like. Fire up this code and read the spec in the time it takes to load:
# download the file from https://dl.fbaipublicfiles.com/LVIS/lvis_v1_train.json.zip
# and unzip it to the current directory
import pandas as pd
import json
with open("lvis_v1_train.json") as fp:
lvis_training_instances = json.load(fp)
print(list(lvis_training_instances.keys()))
# prints out ['info', 'annotations', 'images', 'licenses', 'categories']
categories_df = pd.DataFrame(lvis_training_instances["categories"])
categories_df
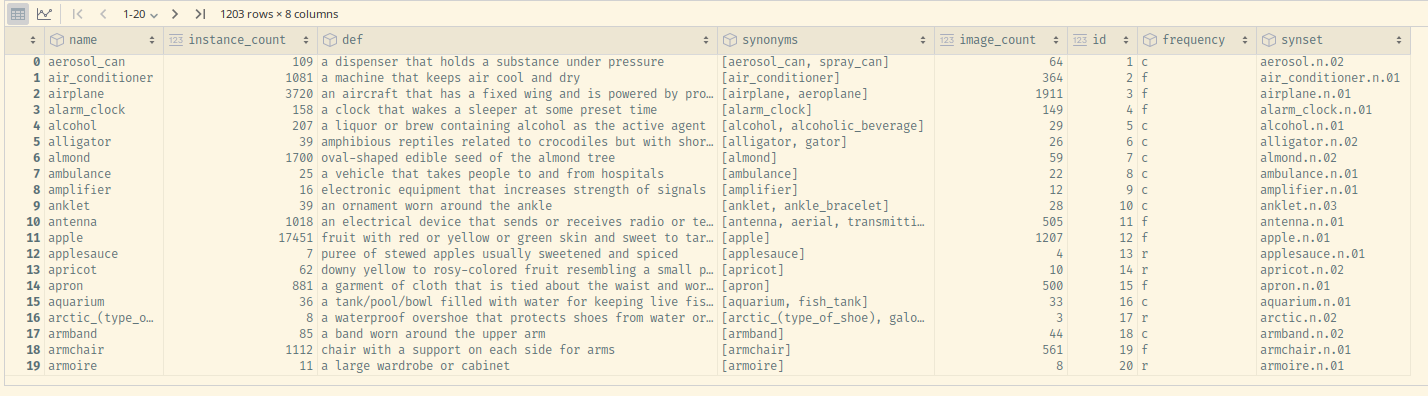
Feel free to explore both the names and the synonyms of the categories – the latter obtainable via a snippet like the following one[3]:
category_names_synonyms = categories_df['synonyms'].explode().unique()
It will become quickly apparent that the problem lies in, well, the problem domain of the model – actual photographs of real-world objects. MechWarrior: Online is pretty far from being photorealistic (both due to stylistic choices and age), so the scenes in the screenshot can’t always be meaningfully interpreted by the model in the context of its training data, even down to basic visual features. Demonstrating the latter problem is the following query, attempting to capture the red landing lights visible throughout the screenshot:
classes = ["red light", "red lightbulb", "landing light"]
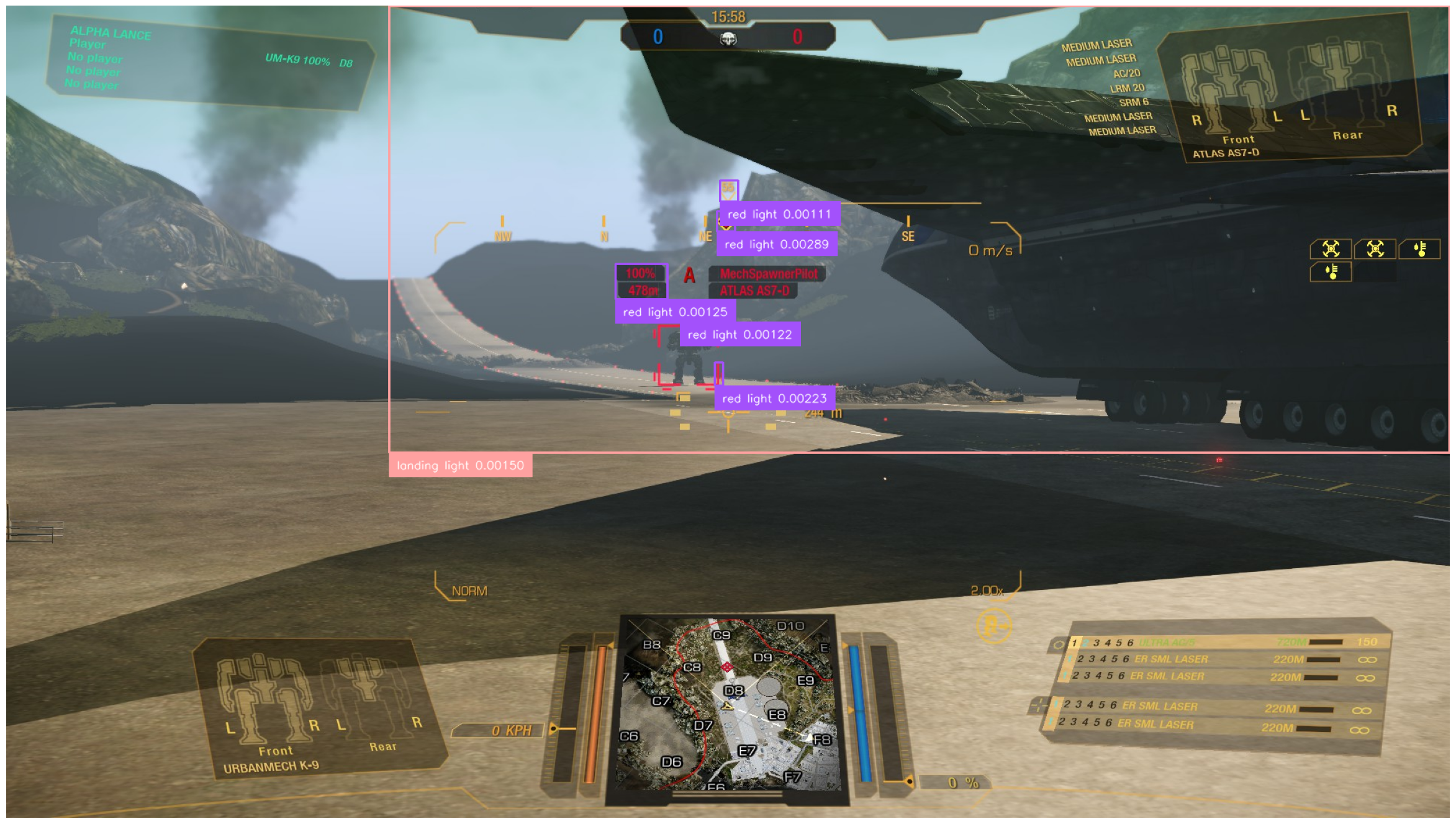
None of the results remotely capture what we intended. The "big" detection is likely due to an association with a… related concept, which we can verify by changing the classes appropriately:
classes = ["airplane", "shuttle", "vehicle"]
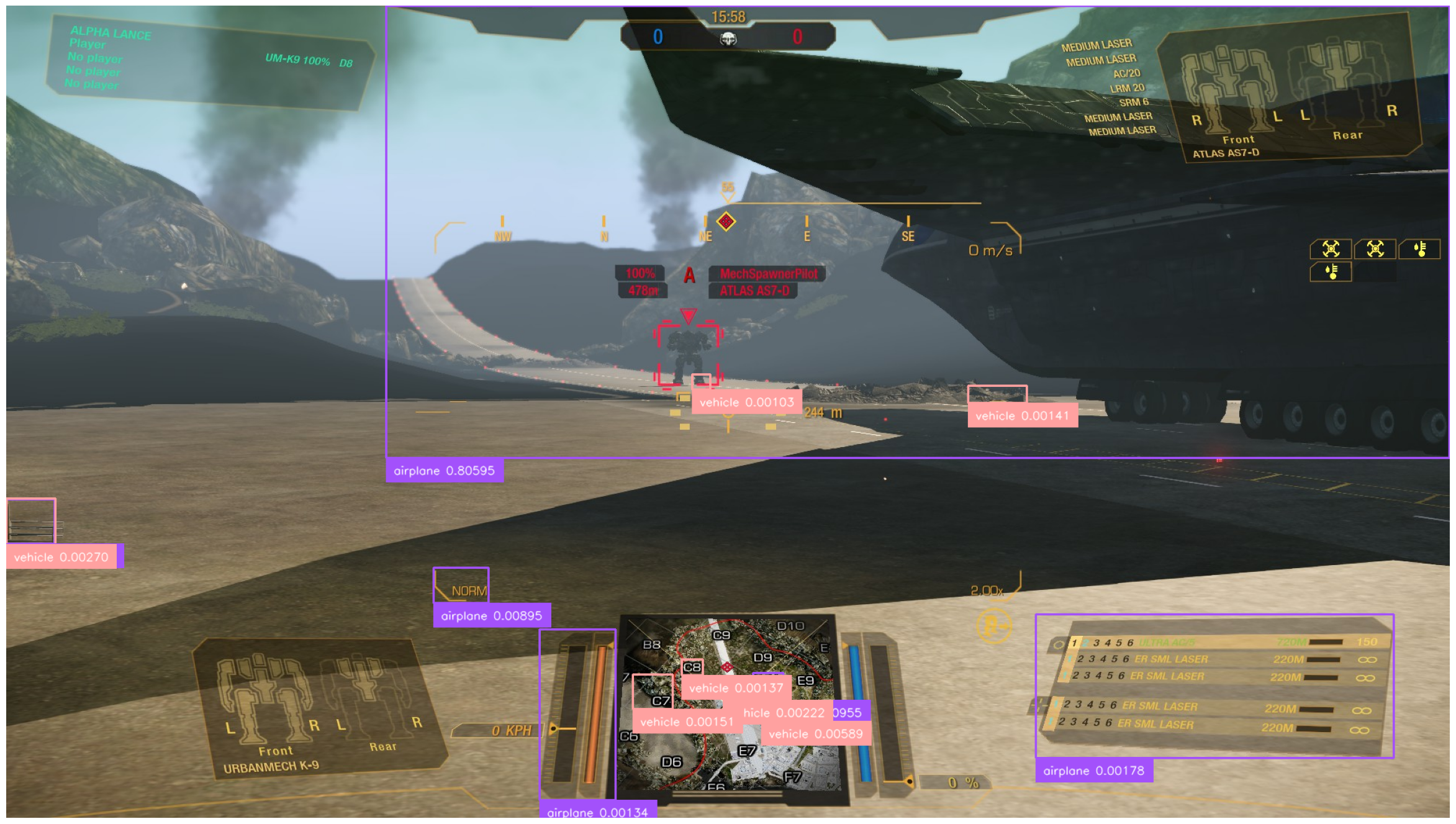
Yep, the model actually does manage to "recognize" the DropShip visible in the screenshot as an airplane[4]. Note the considerably higher confidence – 0.8 is something on the level you would expect from an "actual" detection, as opposed to the unusually low confidence we used for the investigation.
Evidently, YOLO-World is actually capable of detecting artificially generated visuals, but not what we require[5]. In fact, we’ll see similar trends with other SotA (and non-SotA) models: virtually always, training datasets include COCO, Object365, and so on. The "mainstream" models are, in general, not prepared to operate on rendered images, at least not specifically so.
So what can we do with this? One way to go is to adapt the model to our needs.
…and this is what we would have proceeded with, had we not already declared that the blog entry will not be overtly technical. Instead, we’ll perhaps revisit the adaptation task in another entry, but, for now, we’ll just chalk this up as a lesson that even "generalist" models aren’t general enough for each and every use case – context still matters.
Local Large Models to the rescue?
Fortunately, we still have several "levels" to go up on, the first one being trying out a more powerful, but still locally runnable[6], model.
Moondream, the one we’ll use, is described as a "Vision Language Model". Its inputs are both an image, and a text prompt. The latter can be anything from a simple "describe this image" request, to "what is wrong with this specific object in the picture" quasi-anomaly-detection. In between, we have an option to request detection bounding boxes for objects described in a freeform manner, and this is what we’ll use.
As already alluded to, the model is relatively small for an LLM/VLM, and can easily be run on even a laptop-grade GPU, while still having decent time performance. The model is available on Hugging Face, which makes getting it work a breeze. Curiously, there’s little more information about it; publication-wise, the only references that can be found to the model come from comparison papers, such as this one[7].
Moondream: initial approach and examination
Regardless, let’s get right to working on it, following the example code and our test screenshot, with some small modifications:
from transformers import AutoModelForCausalLM, AutoTokenizer
from PIL import Image
# if GPU
DEVICE = 'cuda'
# uncomment if GPU insufficient
# DEVICE = None
model_id = "vikhyatk/moondream2"
revision = "2024-08-26"
model = AutoModelForCausalLM.from_pretrained(
model_id, trust_remote_code=True, revision=revision, device_map=DEVICE
)
tokenizer = AutoTokenizer.from_pretrained(model_id, revision=revision)
base_image = Image.open('blog/img/intro_interface_demo_raw/frame_5.jpg')
def infer(image, question):
enc_image = model.encode_image(image)
return model.answer_question(enc_image, question, tokenizer)
print(infer(base_image, "Describe this image."))
This will give us the following:
The image shows a screenshot from a video game, featuring a player’s view of a futuristic environment with a large vehicle, mountains, and a control panel with various game elements.
Impressive, isn’t it? Five years ago, models of this apparent analytical complexity, running on local hardware, would still be considered science-fiction.
To cut the optimistic tone somewhat, note that we have little amounting to specifics – just a general description of the scene. The output does mention the hills in the background, and a vehicle, but that’s about it.
Wait a minute 'though: maybe the "vehicle" is what we need? To figure it out, we need to write a simple function that annotates the image with bounding boxes resultant from the model’s output. And yes, the model is capable of outputting bounding box coordinates. Here’s the code:
import ast
import numpy as np
import supervision as sv
def bbox_to_annotation(image, prompt):
bbox_str = infer(image, prompt)
# this is unsafe in general, especially when parsing
# open-ended model outputs! Used here for demo purposes only.
bbox = ast.literal_eval(bbox_str)
# retrieve the xyxy coordinates
x1, y1, x2, y2 = bbox
# the coordinates are relative to the image size,
# convert them to pixel values
x1, x2 = (np.array([x1, x2]) * image.size[0]).astype(int)
y1, y2 = (np.array([y1, y2]) * image.size[1]).astype(int)
# create a Supervision Detections object with just our single bounding box
detections = sv.Detections(np.array([[x1, y1, x2, y2]]), class_id=np.array([0]))
# set up the annotator, which is a Supervision API for convenient
# drawing, swapping, and composing various annotation types
annotator = sv.BoxAnnotator()
# need to copy the image – annotator works in-place by default!
return annotator.annotate(image.copy(), detections)
And here’s the invocation with the result:
bbox_to_annotation(base_image, "Bounding box of the vehicle.")
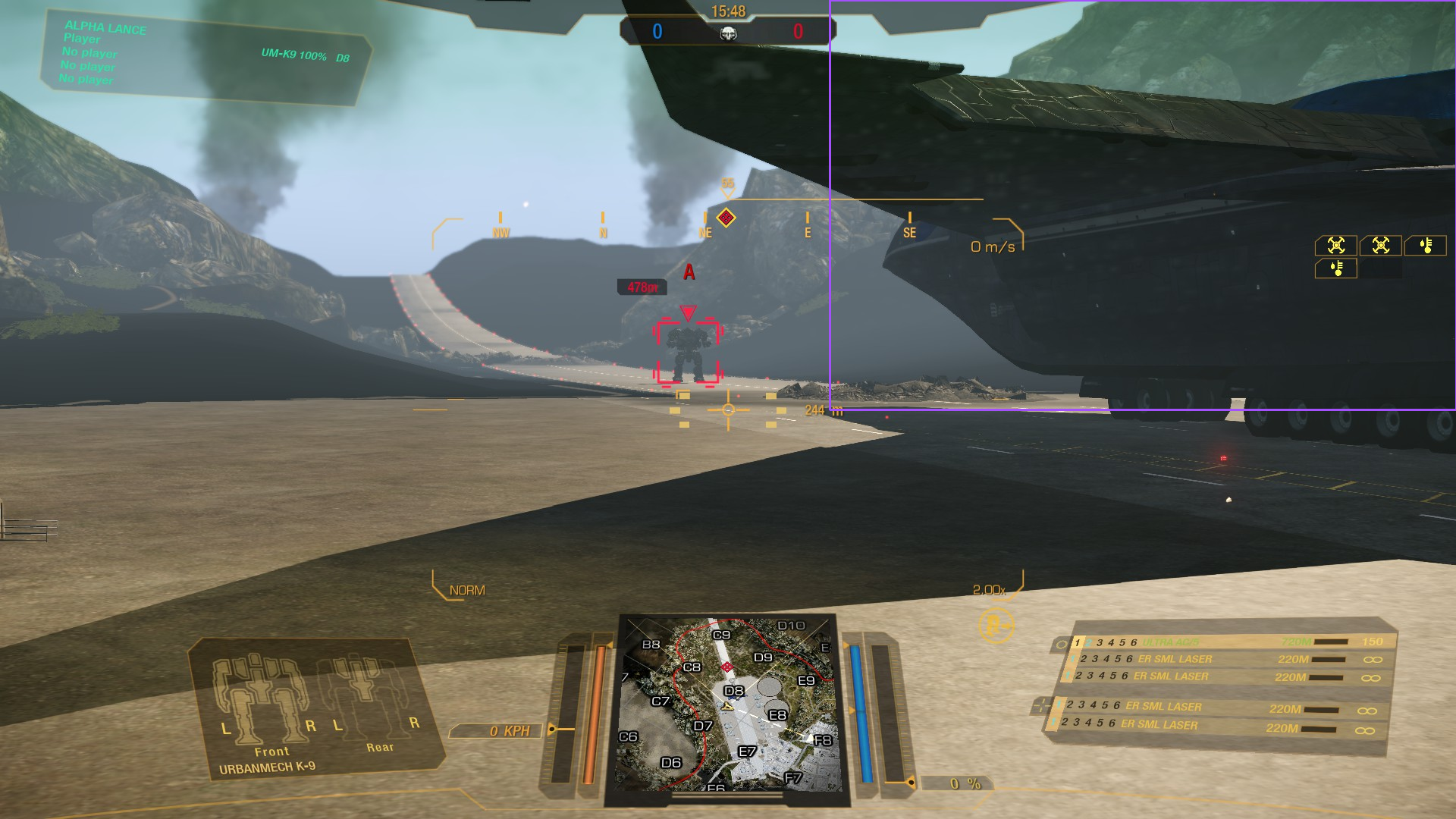
The "vehicle" turned out to be the large DropShip craft sitting on the runway, so that' a miss.
Let’s continue by exploring what the model "sees" in the vicinity of the target designator, as this might give a better idea of what vocabulary we might have to use for a successful prompt. First, directly, by cropping the image to just the designator.

designator_location_base_image = [860, 418, 955, 512]
base_image_designator_crop = sv.crop_image(base_image, xyxy=designator_location_base_image)
infer(base_image_designator_crop, "Describe the image")
The image features a robot with a dark silhouette, standing in a red-framed area. The robot appears to be in a defensive stance, possibly ready to attack.
Apart from the actually internally consistent, but still amusing expression of "defensive stance, […] ready to attack", two observations are of note here:
-
the model does seem to actually recognize mechs as "robots", which is impressive in and of itself;
-
crucially for us, it also notices a "red-frame area", i.e., our designator.
We’ll eventually return to the former, proceeding now with the latter. We know that the model is able to associate the relevant UI element with text embedding in its encoding space that results in the "red-frame". We should now determine how sensitive the model is to this "stimulus" when given a broader context. To do that in a low-tech fashion, we’ll repeatedly run the inference on an image consisting of the designator, plus a variable bit of margin. The variability will span from a relatively small dilation factor of several pixels, up to most of the image in question.
The code to perform the task is pretty simple:
def random_crop(image, base_bb, offsets_range_max: int):
# convert to np.array to allow for vectorized operations
bb_xyxy = np.array(base_bb)
# define the "directions" in which the offsets are applied
# first corner should go only "up" and "left",
# second corner should go only "down" and "right"
offset_direction = [-1, -1, 1, 1]
# generate the random offsets for all BB coordinates
offsets = np.random.randint(0, offsets_range_max, size=len(base_bb))
# perform the "widening" calculation on the original BB
crop_box = bb_xyxy + offset_direction * offsets
# ensure the resultant crop BB is within the image's bounds
repeat_max_by = len(crop_box) // len(image.size)
clipped_crop_box = np.clip(crop_box, 0, list(image.size) * repeat_max_by)
return sv.crop_image(image, clipped_crop_box)
Here’s an example of invocation and result:
random_crop(base_image, designator_location_base_image, 100)
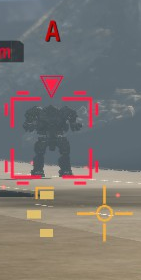
Using the function we just defined, we can now generate the descriptions in the manner we discussed with the following code:
import pandas as pd
from tqdm import tqdm
def extract_descriptions(
source_image,
designator_xyxy,
min_offset=1,
max_offset=1000,
interval=10,
num_iter=10,
):
description_data = []
for offset in tqdm(range(min_offset, max_offset, interval)):
for iter in range(num_iter):
cropped_image = random_crop(source_image, designator_xyxy, offset)
desc = infer(cropped_image, "Describe the image.")
description_data.append(
{"max_offset": offset, "size": cropped_image.size, "description": desc}
)
return pd.DataFrame(description_data)
description_df = extract_descriptions(base_image, designator_location_base_image)
Here is the CSV file of the results obtained after an example run.
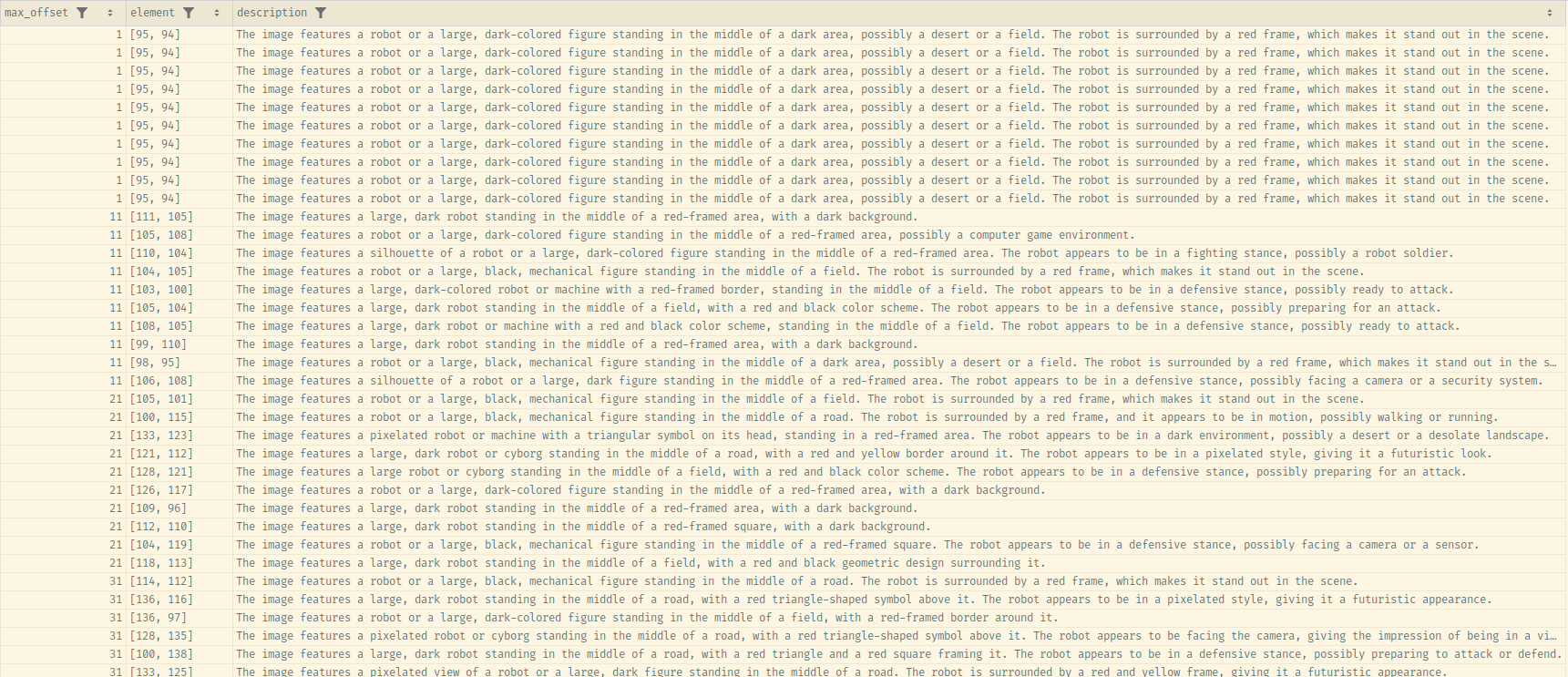
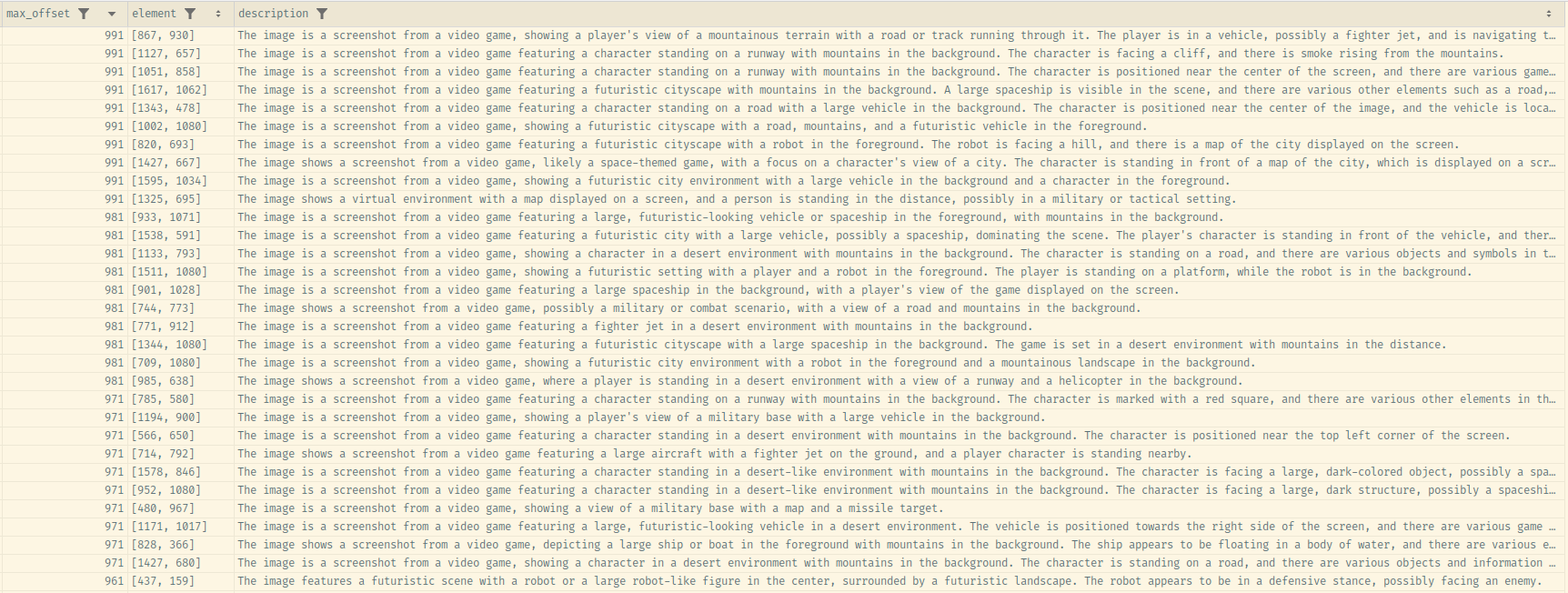
Looking over the data, it is clear that the model recognizes the designator as some element, only for its importance to fall of the description inclusion threshold it in the context of the larger picture.
Regardless, it seems that the model recognizes the frame as a "red" something, as evidenced by this diagram:
import seaborn as sns
_SEARCH_FOR = "red"
# filter the rows that contain the search term
max_offset_with_red = description_df[
description_df["description"].str.contains(_SEARCH_FOR)
]["max_offset"].rename(f"max_offset_{_SEARCH_FOR}")
# plot against all max offsets
sns.histplot(
[max_offset_with_red, description_df["max_offset"]],
palette=["red", "#bbbbbb"],
bins=10,
)
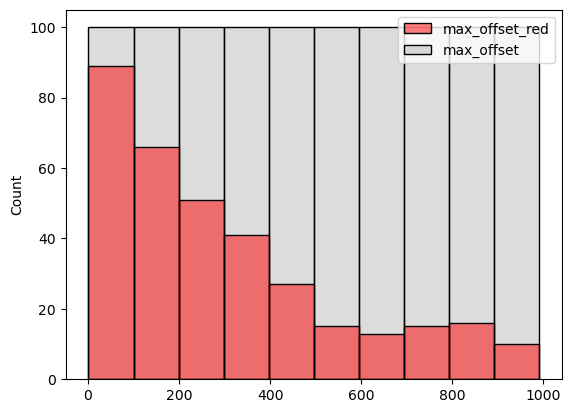
max_offset
values where the word "red" is contained in the result. Note how the frequency of the word’s presence decreases with max_offset
's value – in other words, with the size of the visible area around the designator.Let’s run some aggregation now, so that we may see some trends in the output. We’ll proceed with that by processing the descriptions into a quasi-canonical form, and find the word "red" in the description, along with two neighboring words on each side, and then group the results. For the NLP processing, we’ll use spacy, the documentation of which contains a very nice usage primer, also explaining some basic NLP concepts.
from functools import partial
from typing import Optional
import spacy
_RED_VICINITY = 2
_SEARCH_FOR = "red"
_FIELD_DESCRIPTION = "description"
nlp = spacy.load("en_core_web_sm")
def word_neighborhood(
source: str, lemmatized_word: str, neighborhood_size: int
) -> Optional[str]:
"""Takes a single description, runs basic NLP to obtain lemmatized sentences, and extracts
`neighborhood_size` words around the `lemmatized_word`, including the latter."""
# run the basic NLP pipeline
doc = nlp(source)
try:
# we assume there's only one sentence that has the word
# not a fan of exception-driven logic, but it's cleaner in this case
word_sentence = [
s for s in doc.sents if any(t.lemma_ == lemmatized_word for t in s)
][0]
except IndexError:
return None
# get the lemmatized version of the sentence, without
# stopwords and punctuation
processed_sentence = [
t.lemma_ for t in word_sentence if not t.is_stop and t.is_alpha
]
word_pos = processed_sentence.index(lemmatized_word)
# an alternative would be to use the various Matcher facilities in spaCy,
# but the chosen approach is a bit less cumbersome, doesn't require as much
# knowledge of spacy to read, and we don't care for efficiency in this case
return " ".join(
processed_sentence[
max(0, word_pos - neighborhood_size) : word_pos + neighborhood_size + 1
]
)
def process_description(description_df: pd.DataFrame) -> pd.DataFrame:
# apply the word neighborhood function to the description column
with_red_vicinity = description_df.copy()
with_red_vicinity[_FIELD_DESCRIPTION] = with_red_vicinity[_FIELD_DESCRIPTION].apply(
partial(
word_neighborhood,
lemmatized_word=_SEARCH_FOR,
neighborhood_size=_RED_VICINITY,
)
)
# group by description and aggregate the other fields
return (
with_red_vicinity.groupby(_FIELD_DESCRIPTION)
.agg(
{
"max_offset": np.median,
"size": list,
# Add other fields as needed
}
)
.reset_index()
)
with_red_vicinity_unique = process_description(description_df)
with_red_vicinity_unique
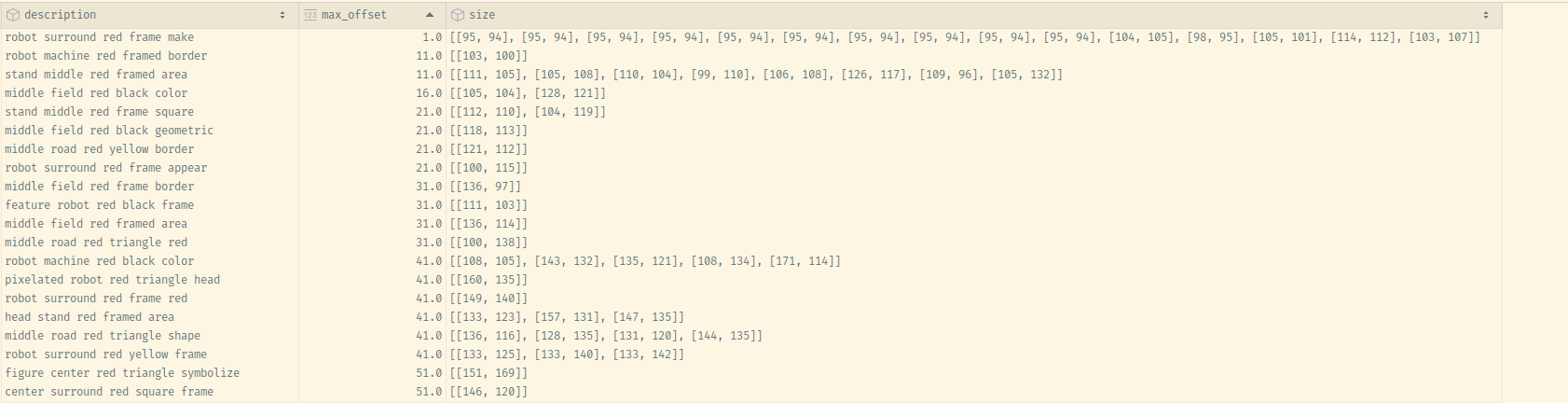
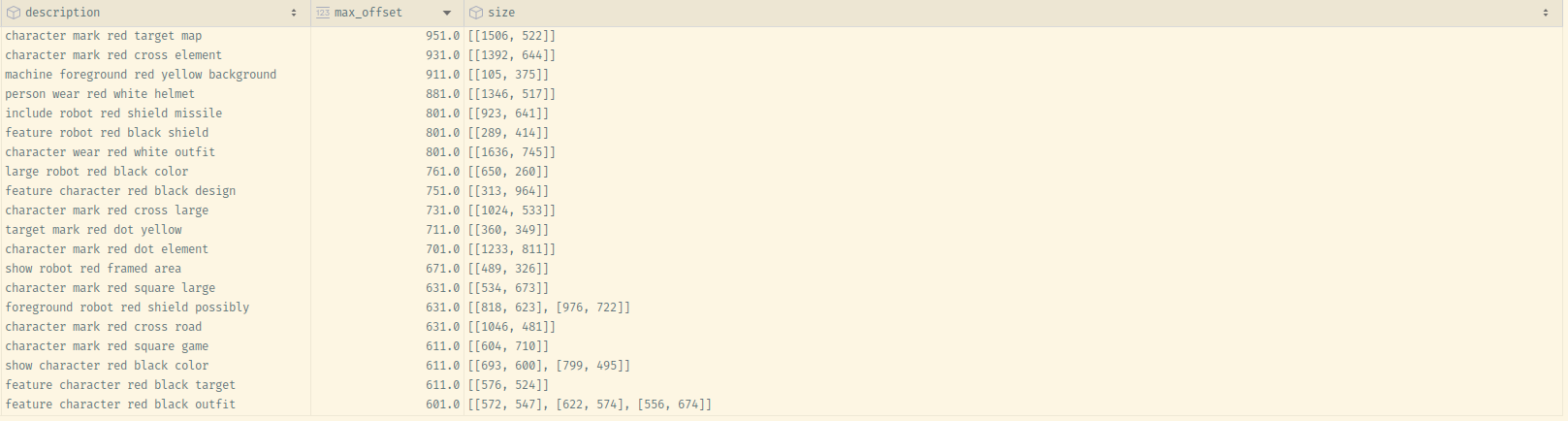
Unfortunately, we confirm the trend we were seeing in the "raw" data – the designator becomes less "distinct" in larger inputs.
In other words, we can try to use the "small detail" text for our BB prompt, but we will not get expected results:
bbox_to_annotation(base_image, "Bounding box of the red square frame border.")
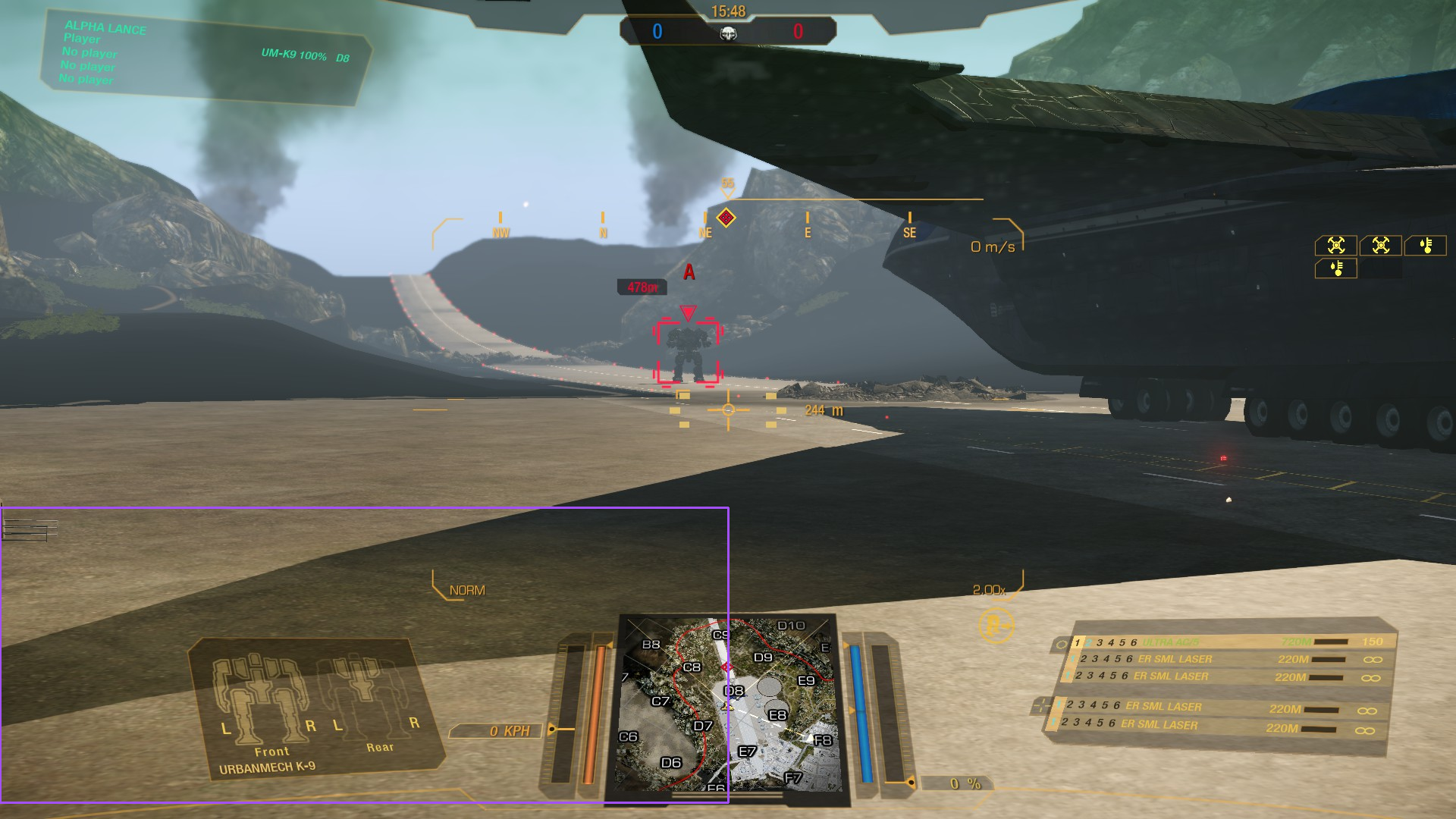
"Prompt engineering" of input images
An alternative way for us to make it easier for the model to "focus" on what we want is to limit the information available in the scene. To do that, we’ll use the insights from the previous entry to simply color-threshold the input image.
Specifically, we’ll mask everything below the 90th percentile of the color threshold for the designators we’ve analyzed, which is color value 177 for the red channel. One way to do this would be to use OpenCV[8]. Here are the relevant code snippets and inference results:
import cv2 as cv
def mask_red_channel_opencv(image, threshold=177):
# using the inRange function, which is slightly more readable than
# creating a mask just based on numpy operations, i.e., something like this:
# mask = (image[:, :, 2] >= threshold).astype(np.uint8) * 255
mask = cv.inRange(image, (0, 0, threshold), (255,) * image.shape[-1])
return image & np.expand_dims(mask, axis=-1)
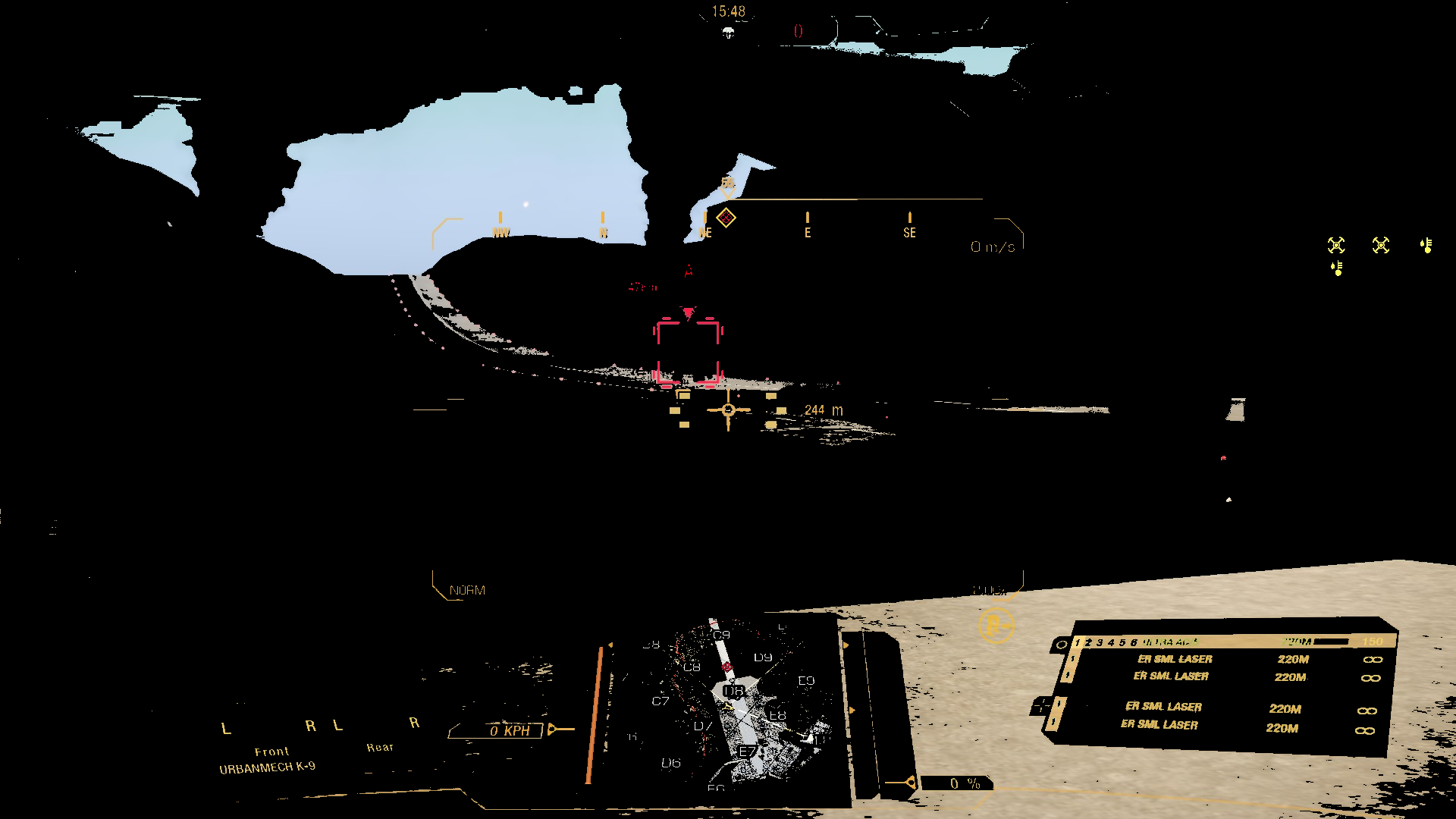
infer(masked_image, "Describe the image.")
The image is a screenshot from a video game featuring a dark background with various elements and graphics. It appears to be a screenshot from a space shooter game, possibly from the "URBANMECHE K-9" series.
bbox_to_annotation(
masked_image,
"Bounding box of the small red frame area.",
)
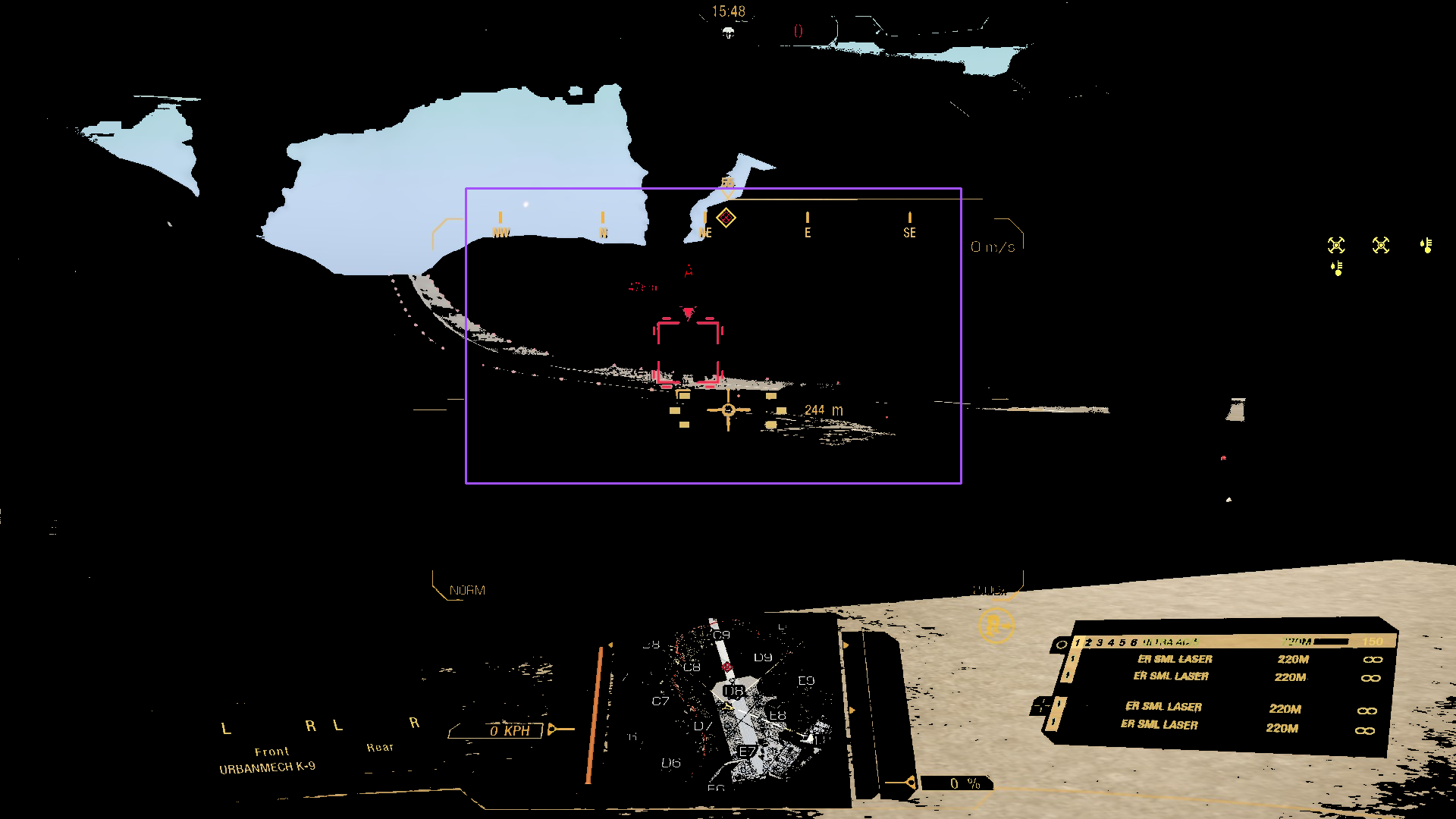
That’s more like it, but still, not perfect. Moreover, the base image is pretty much the "ideal" screenshot. For a more busy scene…
masked_image_busy = sv.cv2_to_pillow(
mask_red_channel_opencv(sv.pillow_to_cv2(busy_image))
)
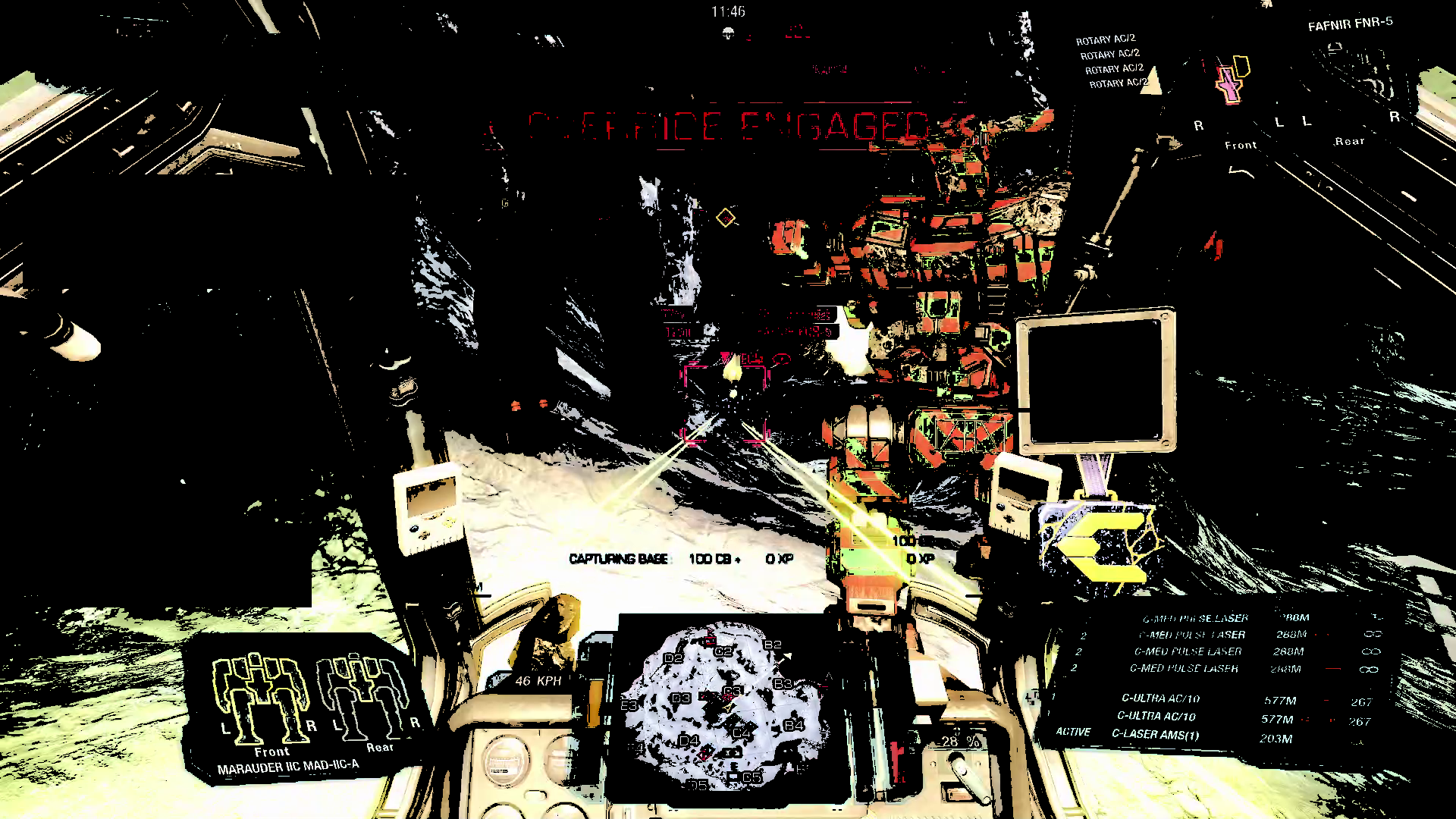
infer(masked_image_busy, "Describe the image.")
The image is a screenshot from a video game featuring a space theme, with various elements of a spaceship cockpit and a cityscape in the background.
bbox_to_annotation(
masked_image_busy,
"Bounding box of the small red frame area.",
)
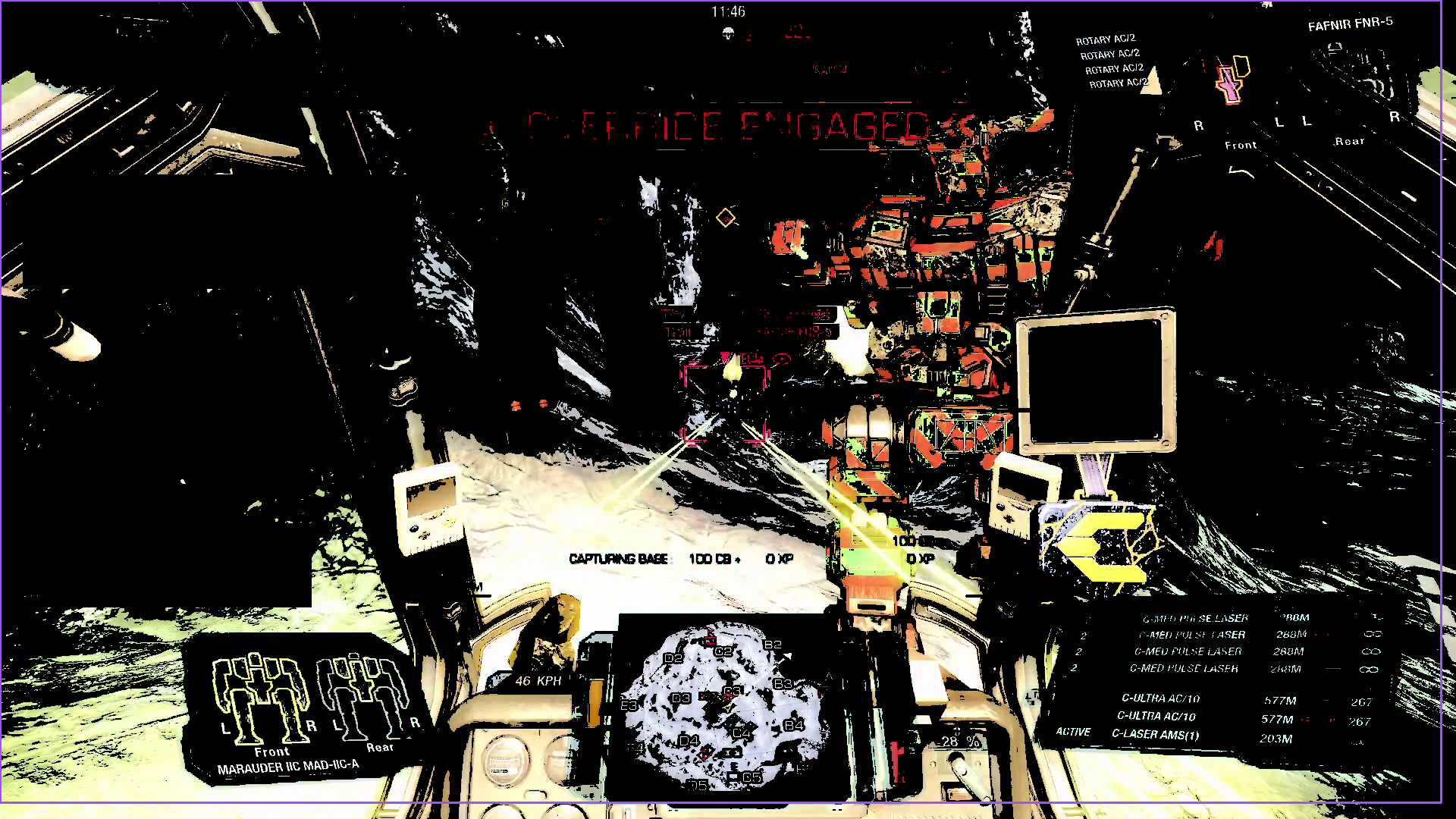
No dice here, either.
Conclusions for Moondream
Like with YOLO-World, it looks like there’s simply a mismatch between what the model was trained to detect, and what we want from it. Perhaps there is a specific prompt that does let the model identify the target designator flawlessly. I’m sure that if there is, I will be made aware of it in 5 minutes of posting this blog entry. However, that prompt certainly can’t be described as easily discoverable.
Again, similarly to YOLO-World, Moondream can certainly be fine-tuned to output what we want, but that is out of scope for this entry, as defined in the introduction.
It would be remiss to leave our consideration of Moondream, and similar distilled LVMs, at this. One thing must be stressed – the models are considerably more powerful than what we’ve shown so far.
Case in point:
bbox_to_annotation(
busy_image,
"Bounding box of the mech on the left.",
)
bbox_to_annotation(
busy_image,
"Bounding box of the mech on the right.",
)
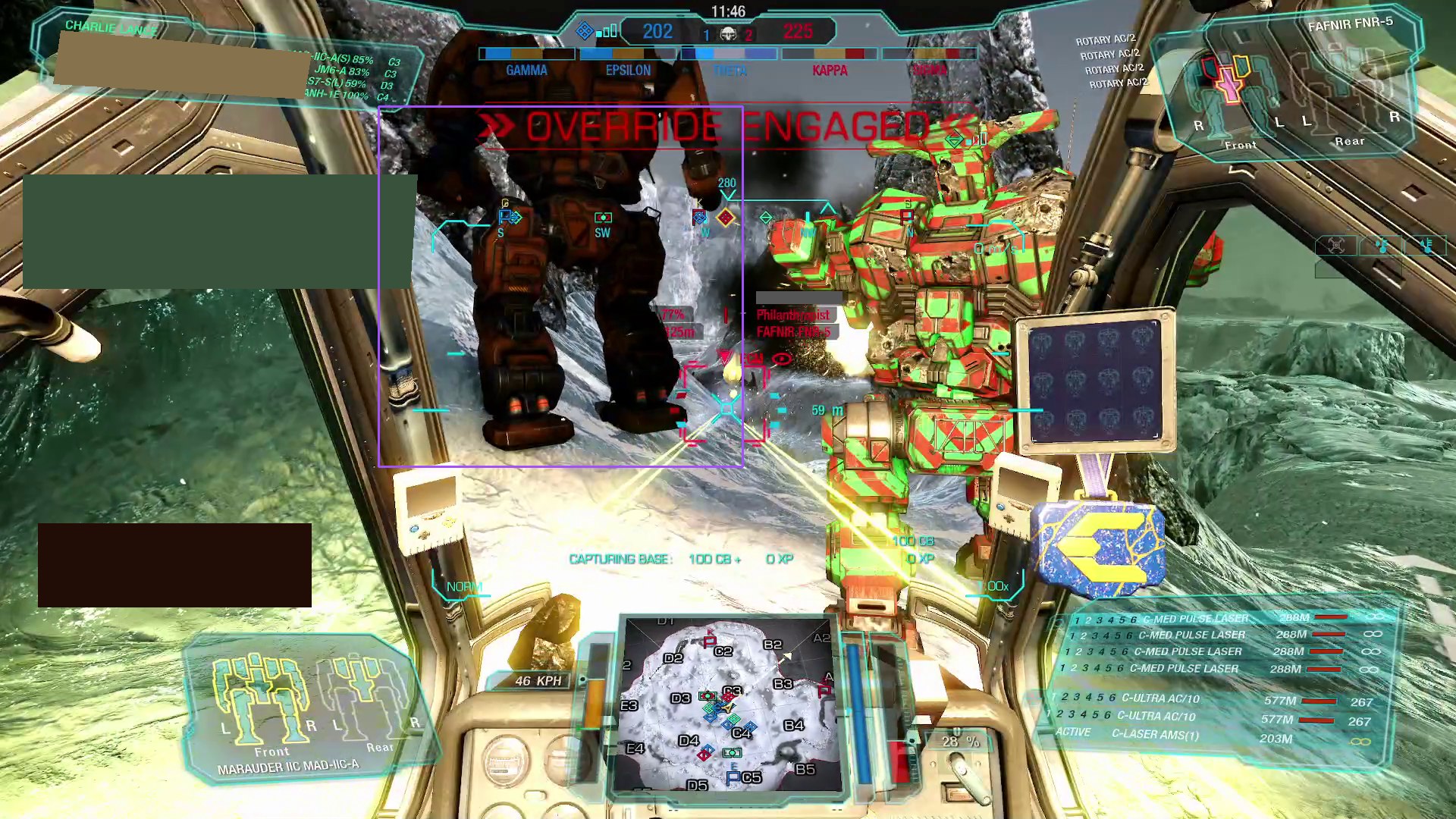
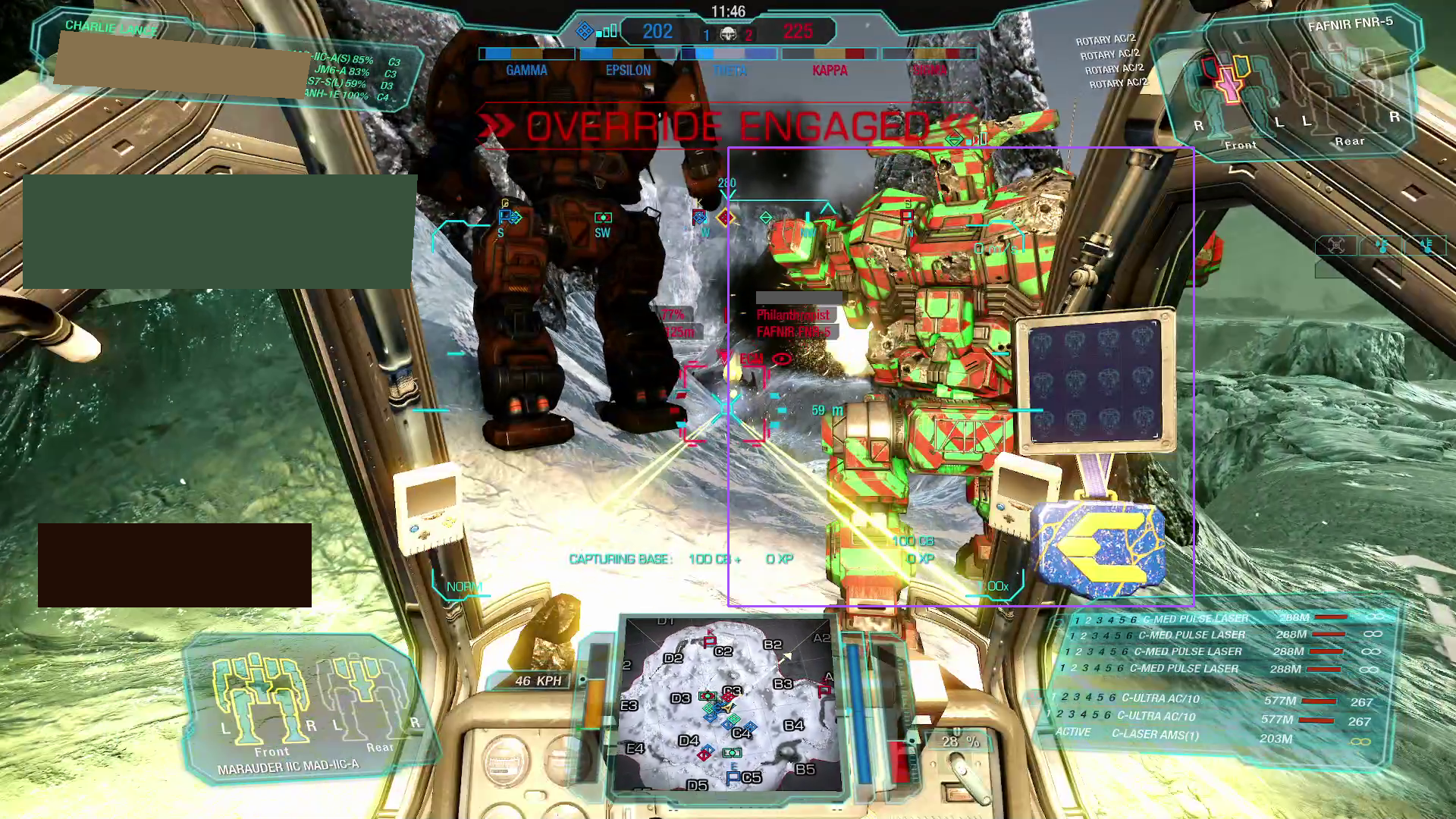
The two prompt results show:
-
Not only does the model readily recognize "robot-like" shapes;
-
it also has an embedding mapping rich enough that it can directly map the phrase "mech" onto these shapes;
-
the detected area actually does roughly correspond to the mechs in the scene.
All this is to say: Moondream, and models like it, are certainly powerful enough to potentially serve our main purpose, i.e., detecting the mechs on the screen, with only some tweaking. We’ll revisit this opportunity space in future entries.
Do we even need the designator? Looking into auto-segmentation via SAM 2
Throughout the current body of the blog series, we’ve operated on an assumption that extracting the designator images is our best bet of building a training set for the "final" mech detection model. Is that necessarily the case?
Those knowing a certain "law" already know the answer. Indeed, one of the changes in recent years has been the appearance of modern generalized image segmentation models[9]. The output thereof can be then used as training data for the target detection models.
We’ll try out a relatively recent one, Segment Anything 2 from Meta (née Facebook), available alternatively on Hugging Face. There’s also a rather impressive landing page, with various demos, including interactive ones.
Perusing the docs, notebooks, etc., we should notice quite quickly what the major trait is of the model, setting it apart from the "classical" segmentation models. Namely, it can provide complete, or near-complete segmentation of an image without any other input, as evidenced by the screenshot below…
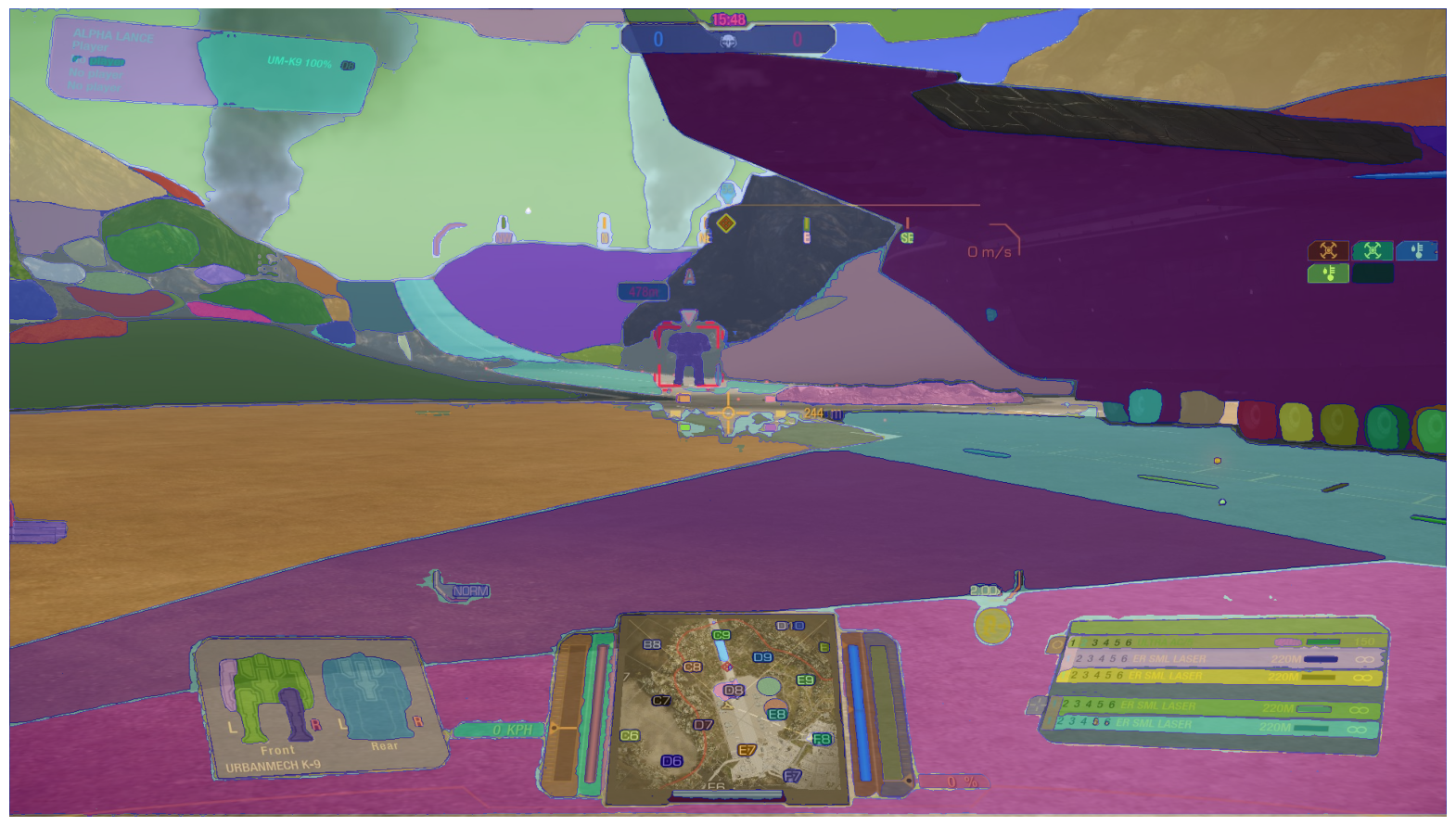
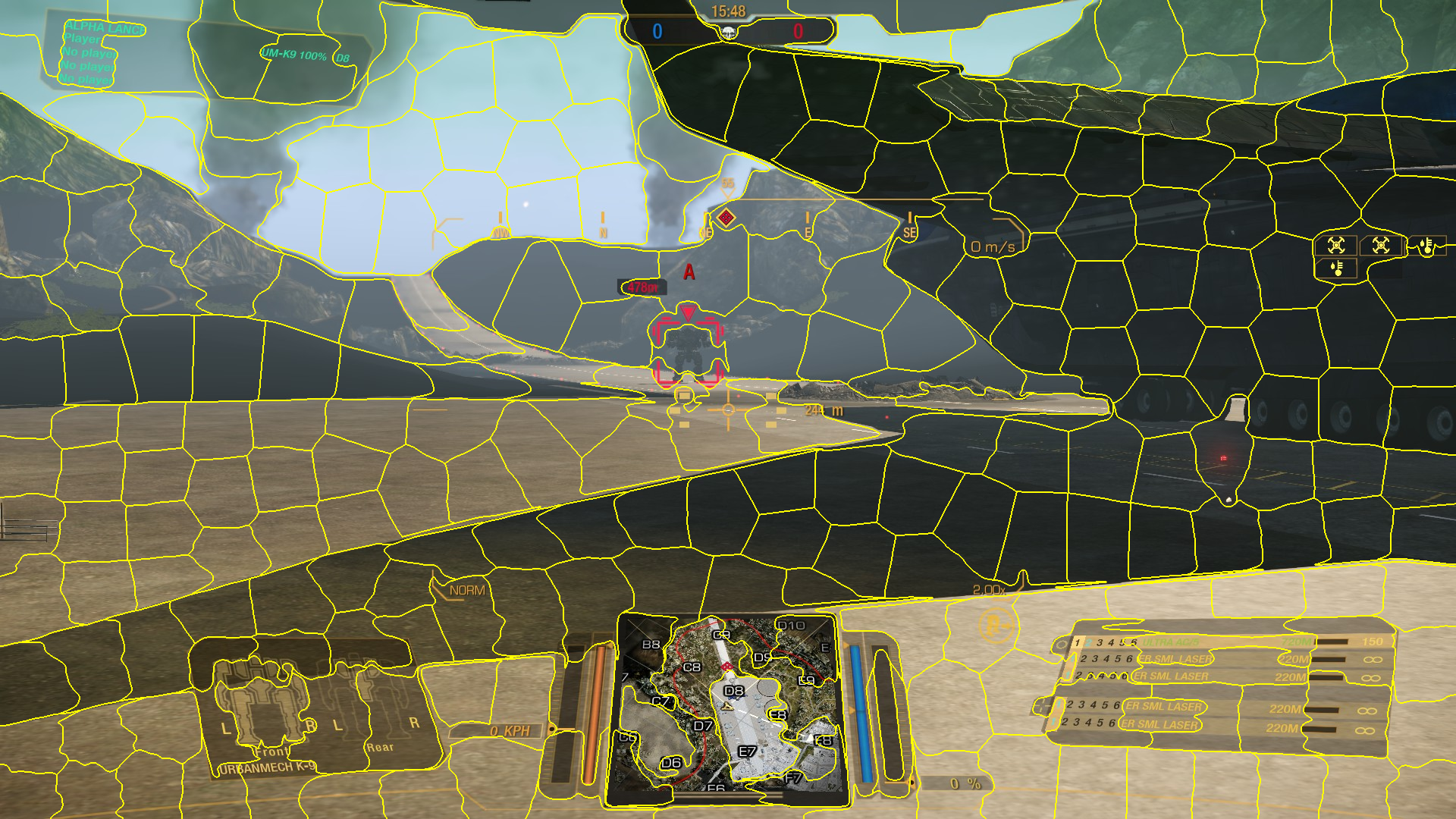
Its real power lies in the ability to segment images based on a point prompt. Well, that and being able to process videos based on that original prompt (and being resilient against occlusions, and other things…).
Let’s see a little demonstration. The code here is heavily derived from Roboflow’s blog entry, authored by Piotr Skalski, on SAM 2, the primary adaptation being generalizing most of the logic into a single-function API[10]:
# again, this is mostly code from
# https://blog.roboflow.com/sam-2-video-segmentation/
# reorganized and slightly amended
import cv2 as cv
import numpy as np
import pandas as pd
import supervision as sv
from pathlib import Path
def get_masks_as_detections(object_ids, mask_logits) -> sv.Detections:
"""Converts SAM 2 output to Supervision Detections."""
masks = (mask_logits > 0.0).cpu().numpy()
N, X, H, W = masks.shape
masks = masks.reshape(N * X, H, W)
return sv.Detections(
xyxy=sv.mask_to_xyxy(masks=masks), mask=masks, tracker_id=np.array(object_ids)
)
def add_points_to_predictor(predictor, inference_state, point_data: pd.DataFrame):
"""Add points to SAM 2's inference state.
This function assumes it receives a DF with the columns:
["point_xy", "object_id", "label", "frame_id"]
where "label" is 1 or 0 for a pos/neg example.
"""
# aggregate by frame and object, to conform to batching modus
# of predictor.add_new_points
aggregated = point_data.groupby(["frame_id", "object_id"]).agg(list)
for (frame_id, object_id), row in aggregated.iterrows():
points = np.array(row["point_xy"], dtype=np.float32)
labels = np.array(row["label"])
predictor.add_new_points(
inference_state=inference_state,
frame_idx=frame_id,
obj_id=object_id,
points=points,
labels=labels,
)
def ensure_frames_generated(source_video: Path, frame_dir_root: Path) -> Path:
"""Checks if the frame images, necessary for SAM 2's state init and processing, are present.
If not, generates and saves them to the corresponding `frame_dir_root` subdirectory.
"""
video_name = source_video.stem
frame_dir = frame_dir_root / video_name
# a simple check - if the video for the directory exists,
# we assume the frames are already generated
if not frame_dir.exists():
sink = sv.ImageSink(target_dir_path=frame_dir, image_name_pattern="{:04d}.jpeg")
with sink:
for frame in sv.get_video_frames_generator(str(source_video)):
sink.save_image(frame)
return frame_dir
def segment_video(
predictor,
source_video: Path,
point_data: pd.DataFrame,
target_video: Path,
label_annotator: sv.LabelAnnotator,
mask_annotator: sv.MaskAnnotator,
frame_dir_root: Path = Path("./video_frames"),
):
frame_dir = ensure_frames_generated(source_video, frame_dir_root)
# init model's state on the video's frames
inference_state = predictor.init_state(video_path=str(frame_dir))
# add segment guidance points to the model
add_points_to_predictor(predictor, inference_state, point_data)
video_info = sv.VideoInfo.from_video_path(str(source_video))
frames_paths = sorted(
sv.list_files_with_extensions(directory=frame_dir, extensions=["jpeg"])
)
with sv.VideoSink(str(target_video), video_info=video_info) as sink:
for frame_i, object_ids, mask_logits in predictor.propagate_in_video(
inference_state
):
frame = cv.imread(str(frames_paths[frame_i]))
detections = get_masks_as_detections(object_ids, mask_logits)
frame_annotated = label_annotator.annotate(frame, detections)
frame_annotated = mask_annotator.annotate(frame_annotated, detections)
sink.write_frame(frame_annotated)
which can be invoked like this:
segment_video(
predictor,
source_video=Path(SOURCE_VIDEO),
point_data=point_data,
target_video=Path(TARGET_VIDEO_PATH),
label_annotator=sv.LabelAnnotator(
color=sv.ColorPalette.DEFAULT,
color_lookup=sv.ColorLookup.TRACK,
text_color=sv.Color.BLACK,
text_scale=0.5,
text_padding=1,
text_position=sv.Position.CENTER_OF_MASS,
),
mask_annotator=sv.MaskAnnotator(
color=sv.ColorPalette.DEFAULT, color_lookup=sv.ColorLookup.TRACK
),
)
The predictor
value is the result of a sam2.build_sam.build_sam2_video_predictor
call, the instructions for setting up which are available in the relevant documentation. In all examples here, we are using the "large" model variant.
Since we’re switching from images to video, we need to actually choose a video snippet. We’ll go with this one:
As you can see, the video can potentially be challenging to segment – the color palette is muted and homogenous, the objects of interest are either small, occluded, or blend with the background well. Nevertheless, let’s try marking a single point – specifically on the very fist visible mech up in front – and see how SAM 2 performs:
That’s actually impressive. The model does manage to keep up with both the objects and the camera’s movement, only "picking up" some extraneous elements, but never extending the mask to an unacceptable level. The only bigger hangup is it losing the object and switching tracking to another one after the zoom level switch – again, nothing concerning, as there’s no indication SAM 2 should be resilient against that.
OK, so we’ve covered a single object, but, in this snippet, a grand total of 5[11] are available to segment. We extend the point data to the following form:
import pandas as pd
# as a reminder, "label" denotes whether
# the example is "positive" or "negative"
#
# we identify our "segmentation tracks"
# by the "object_id" field
point_data = pd.DataFrame(
[
[[1122, 347], 1, 1, 52],
[[1167, 348], 2, 1, 52],
[[1030, 350], 1, 1, 76],
[[880, 338], 2, 1, 113],
[[900, 355], 2, 0, 113],
[[1675, 435], 3, 1, 130],
[[1508, 446], 4, 1, 131],
[[1553, 444], 4, 0, 131],
[[1867, 427], 5, 1, 145],
[[1202, 391], 4, 1, 363],
[[1258, 435], 3, 1, 363],
[[755, 324], 2, 1, 393],
[[1145, 399], 4, 1, 436],
[[1155, 331], 4, 1, 436],
[[1062, 383], 4, 1, 615],
[[1011, 391], 3, 1, 636],
],
columns=["point_xy", "object_id", "label", "frame_id"],
)
For reference and visualization, here is the representation of the example points on the respective frames, as well as the initial segmentation provided by SAM 2’s model state.
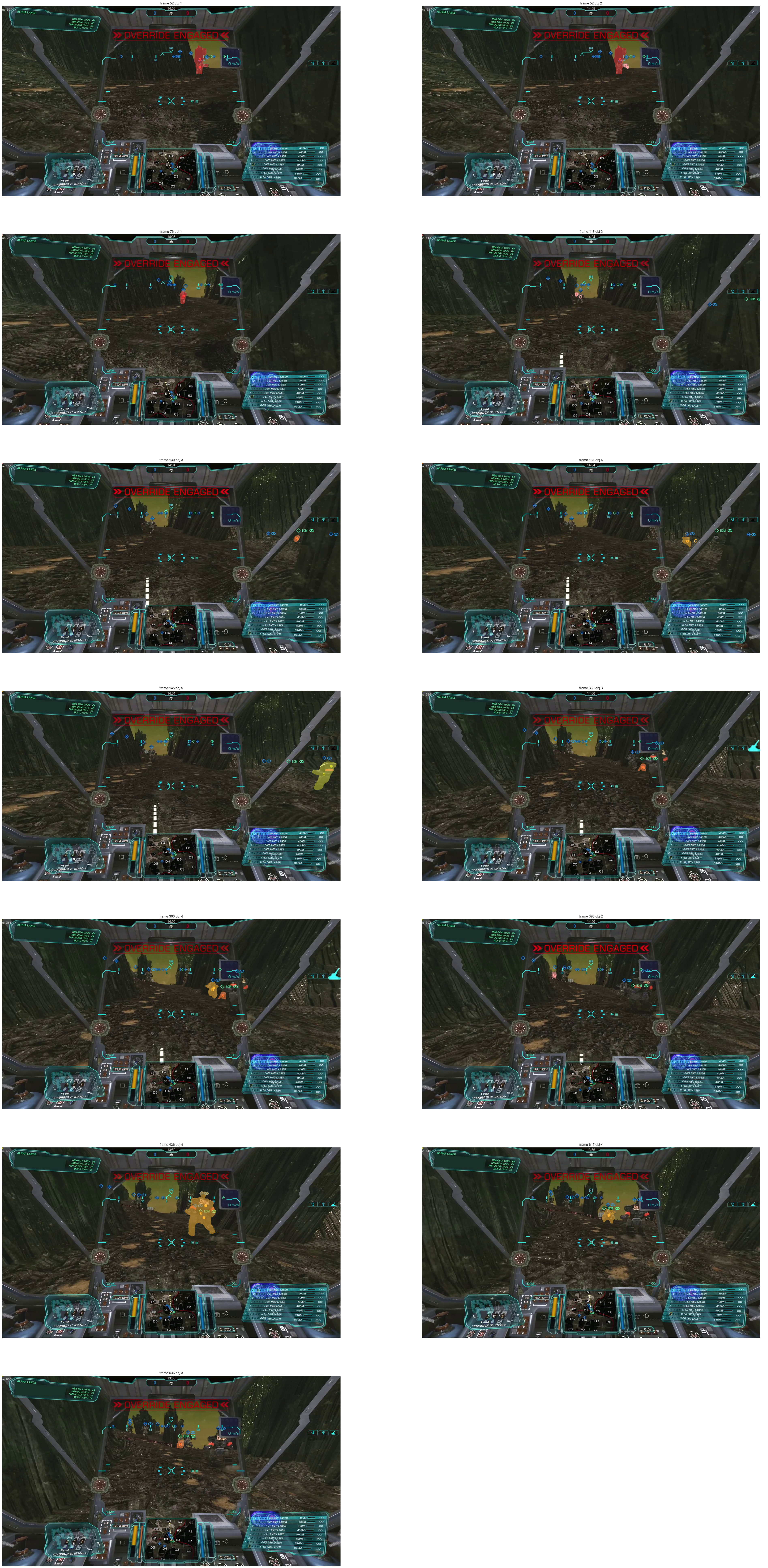
We can see that the static image masking does look promising. The biggest problem is that SAM 2 is a bit "greedy", and often incorporates parts of the background – see especially the first frame presented – hence the need for several negative examples. We also had to provide additional example points for objects that "started out" adjacent, or even overlapping. Again, still a good show so far, given the difficulty of the input video.
From the examples provided, we get the following segmentation results:
Comparing to the single-point example, the most notable phenomenon is a markedly reduced tracking ability of the "original" object (denoted as 0
). This is likely due to attempting simultaneous segmentation on another mech (denoted as 1
) that’s passing right behind 0
. Overall, the model is unable to persistently track virtually any of the objects without further point examples, and even then, it eventually loses persistence. Interestingly, it does manage persist the tracking of one object over the zoom sequence (4
), which means SAM 2’s anti-occlusion facilities are quite powerful.
Nevertheless, we must remind ourselves that we are not investigating SAM 2 for tracking capabilities, but to provide a source of training data for detection. And, for this purpose, it seems like a promising direction. The model produces masks that are almost always more precise than the equivalent of the target designator’s shape (something we haven’t talked about yet – even when we do get the designators, a cleanup step will still be necessary before we proceed with actual training, or fine-tuning, of a detection model).
Additionally, we’ve imposed quite a challenge on SAM 2. There’s plenty of "real world" use cases where the model would be likely to perform with much greater fidelity, and so provide even better annotation data.
The only gripe is resource consumption – even the "tiny" version of SAM 2 uses too much VRAM for most consumer GPUs. The hope lies in the larger community creating derivatives that require less memory, while having similar performance.
"Mainstream" LLMs
While things were a bit different even a year ago (due to lacking image-assisted prompt possibilities), it would now be remiss to not include a comparison of processing through the "mainstream" LLMs. We’re going to take a look at two families of them: Anthropic’s Claude and OpenAI’s GPT-4o.
The plan is as follows – first, we’re going to supply the demo image with the following prompt:
The image is a screenshot of a video game. On the screenshot, there is a target designator, shaped like a square with middle portions of its sides missing, all colored red. Provide the bounding box pixel coordinates of this designator.
We’re going to repeat the same text prompt with the "busy" image. Finally, we’re going to use one of the frame images from the test video, i.e., this one:
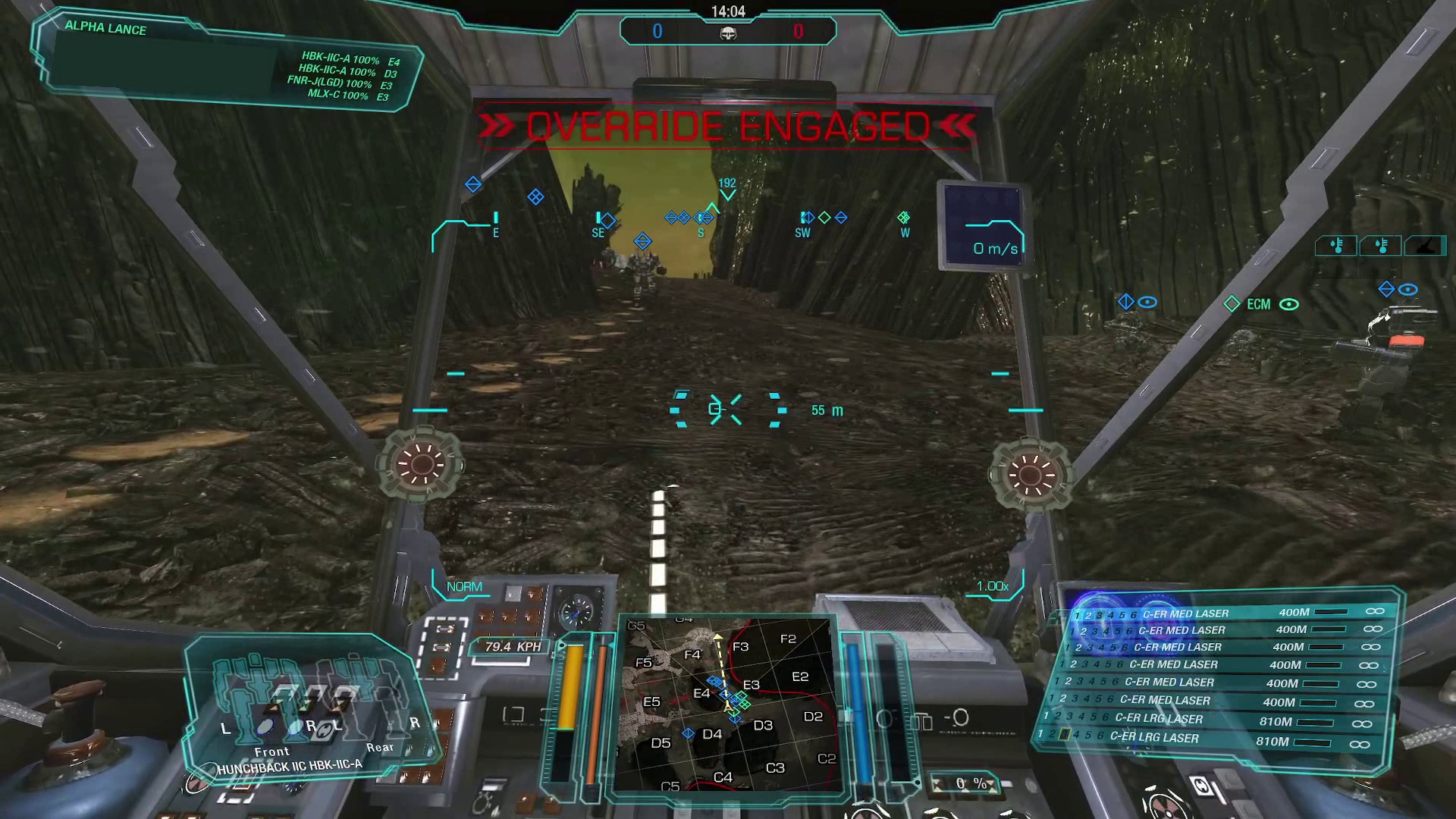
and the prompt:
The image is a screenshot from a video game in the BattleTech/MechWarrior franchise. Provide bounding box locations of all the mechs in the image.
We’ll adapt this preliminary plan in case a potential for additional insights manifests itself.
Claude
Spoiler: this won’t go well. Starting with demo image and the specified prompt:
I apologize, but I’m not able to provide exact pixel coordinates from the image. However, I can describe the location and appearance of the red target designator you’re referring to:
The red target designator is located roughly in the center of the image, slightly below the midpoint vertically. It’s surrounding what appears to be a mech or large robotic figure standing on a road or runway.
The designator consists of a square shape with the middle portions of each side missing, creating a crosshair-like effect. Inside this designator is a distance readout showing "478m".
If you need precise pixel coordinates, you would need to use an image editing tool or specialized software to analyze the image. I can only provide descriptive information based on what I see in the image.
(emphasis added)
So, unfortunately, it looks like Claude immediately turns out to be a dud for our purposes. Now, it’s not all bad – it does recognize both the designator and the mech in the image. We note also that Claude considers the distance readout as being inside the designator; we’ll get back to this observation in the ChatGPT section.
Just not to end Claude’s exploration too abruptly, let’s see what it has to say for some other images and prompts. Here’s what it outputs when provided the "busy" image, with an adapted prompt:
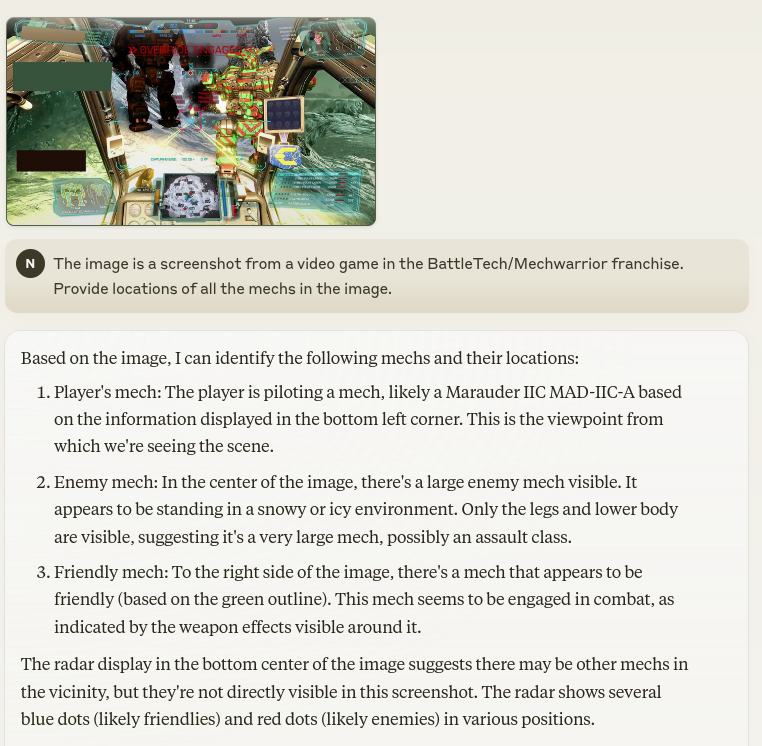
Unfortunately, Claude doesn’t seem to recognize the mech that’s actually being targeted – there was a bit of hope the designator itself would provide sufficient context. At least it does identify the two other relevant objects in view. However, it describes the one on the left erroneously as hostile – quite obviously, due to the "friendly" marker above visually separated from the mech by one of the UI elements.
Interestingly, Claude does appear to have some information about the franchise, as it correctly references the existence of the assault weight class[12]. Not surprising, 'though – BattleTech is enjoying its 40-year anniversary right about now.
Finally, let’s see if Claude can at least recognize mechs in a more challenging screenshot:
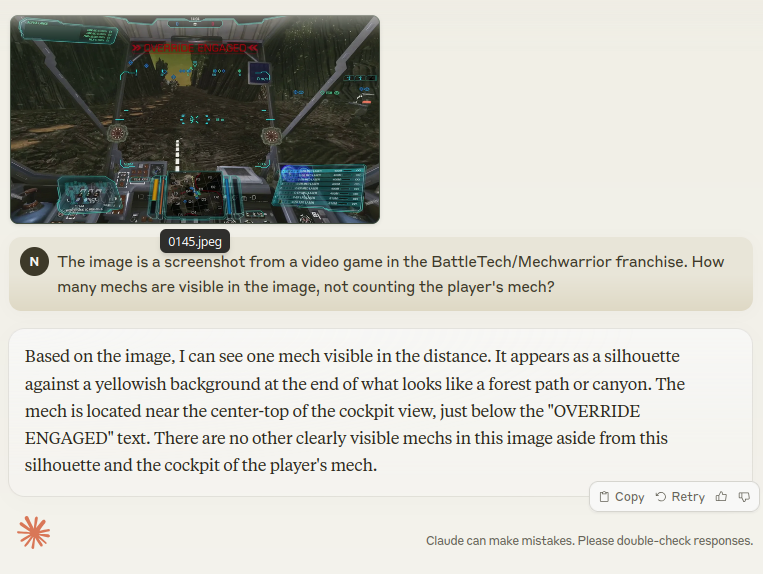
Disappointing as well: the correct answer is 5, not counting the player.
Before we conclude, we must note that the prompts were answered by the free version of Sonnet. While this may seem unfair, Claude all but disqualified itself by refusing to provide bounding box coordinates, apparently by design. Frankly, the author is unwilling to splurge >20€ just to check if the Pro version has a different system pre-prompt.
Otherwise, it’s worth pointing out that Claude, for our use cases, appears to have similar capabilities to a model such as Moondream.
ChatGPT
We’re using GPT-4o, as o1-preview doesn’t seem able to ingest images currently. For the demo image, using the prompt specified in the section intro, the model does provide a bounding box: (810, 370, 960, 520)
. The coordinates translate to the following clip:
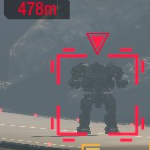
Partially true, but not exact. The model seems to consider the distance readout as part of the designator. This quirk is not only present here but also in Claude and in Moondream. Honestly, not entirely sure what is the reason. The best guess is these sorts of UI elements, (i.e., designators) indeed often have supplementary icons or text "attached" to them, and this is reflected in whatever common data sets were used to train the models. So, for the model, the designator indeed comprises both the element itself and any "add-ons", an effect similar to the well-known "huskies are on snowy background" class of error – if it even can be considered an "error".
A follow-up prompt:
The bounding box provided is too large. It should only contain the designator’s square shape itself. Please refine the coordinates.
yields (842, 406, 927, 491)
:

better, but still not ideal.
Let’s move on to the busy image, where the bounding box is given as (617, 32, 1775, 1055)
:
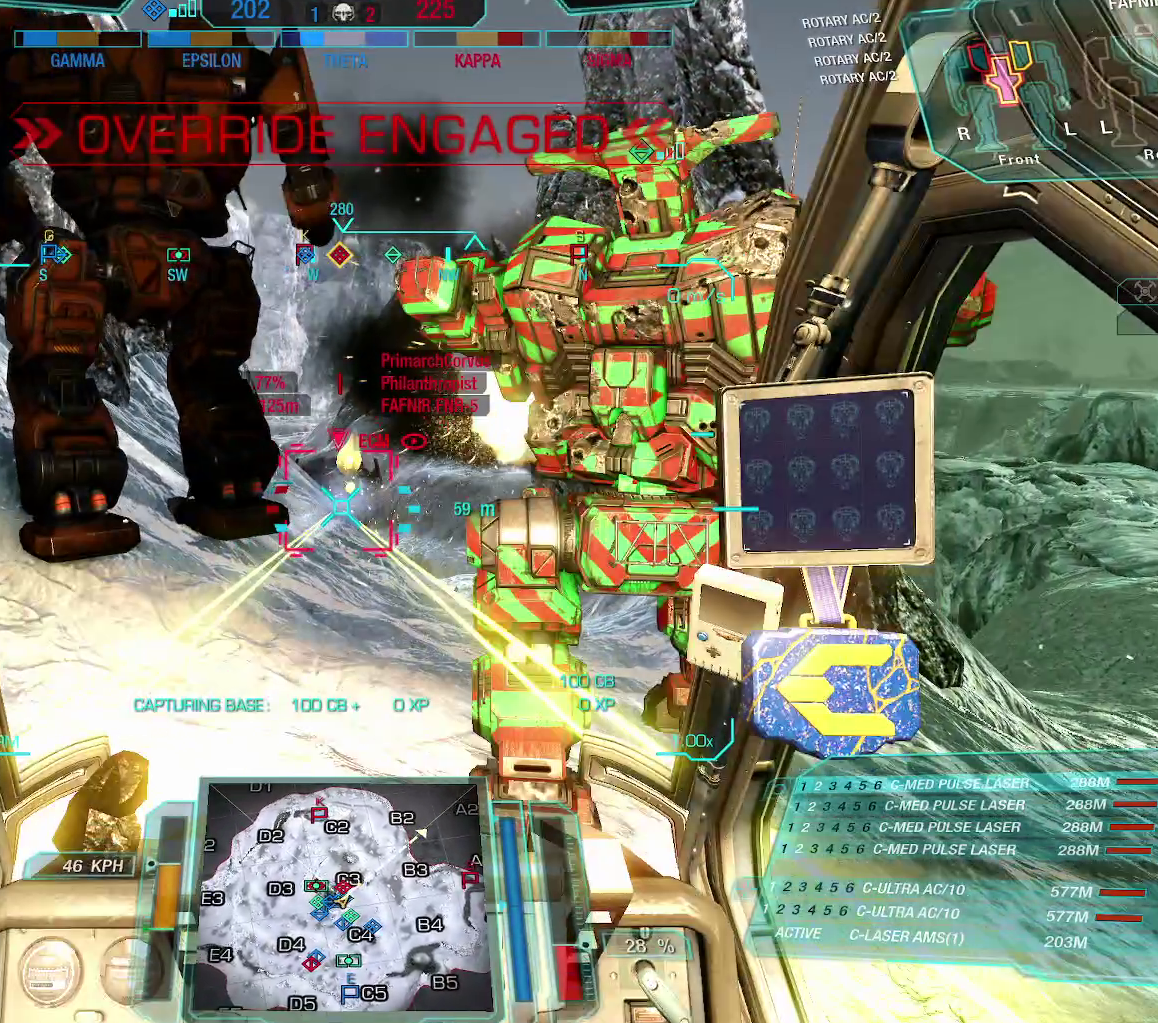
which, as we already guess from the coordinates alone, is way too imprecise – although, in fairness, it does include the designator itself. Funnily enough, the model, when requesting refinement, eventually "gives itself up" with this choice snippet:
It appears that my current attempt still did not yield the correct bounding box for the smaller red designator. Given the complexity of identifying the exact designator in this image, I would recommend a more manual analysis or specialized visual inspection using specific graphic tools. If you prefer, you could manually examine pixel clusters using an image editor to isolate the exact region you’re interested in.
All right, let’s do some follow-ups with "smarter" queries, like we did with Claude.
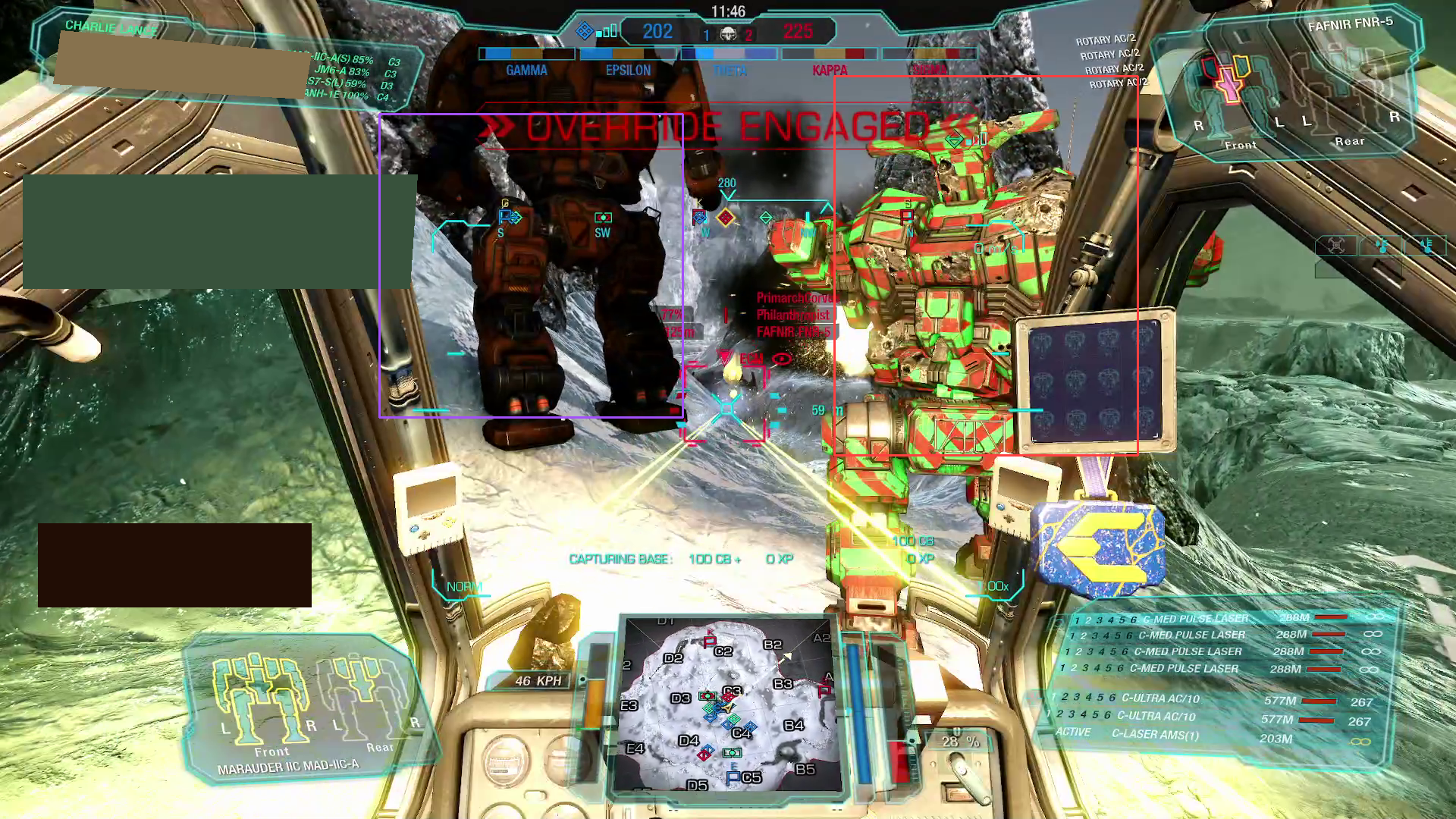
For the "BB location" prompt (the second one from the intro), ChatGPT seems to provide similar results as Moondream. Note that the image above was generated "manually", which is actually not strictly necessary – 4o absolutely can, and will, when asked for, draw the bounding boxes automatically.
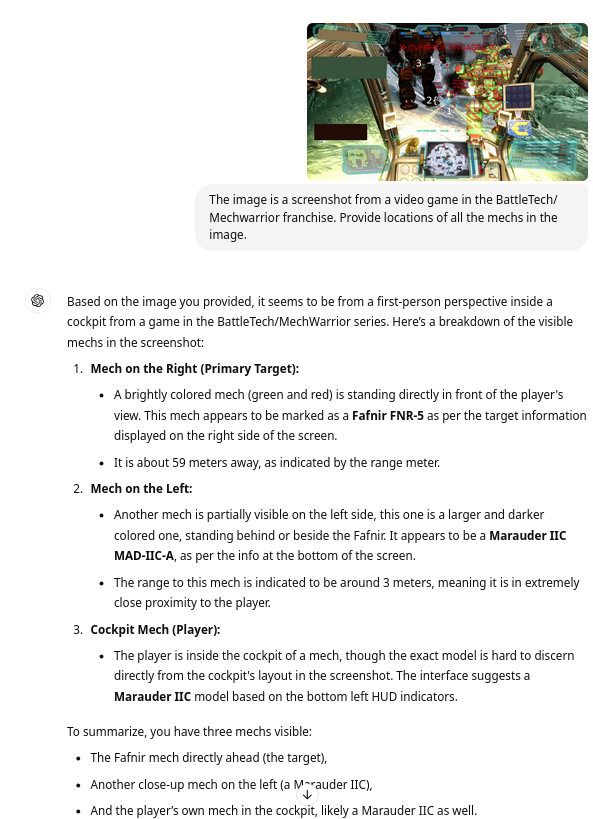
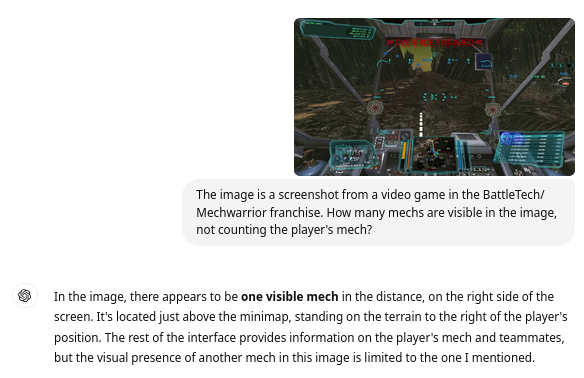
ChatGPT returns similar results as Claude. When pressed with the suggestion of the correct answer (5), and asked to provide bounding boxes, it does so, but hallucinates heavily, and returns completely incorrect BBs[13].
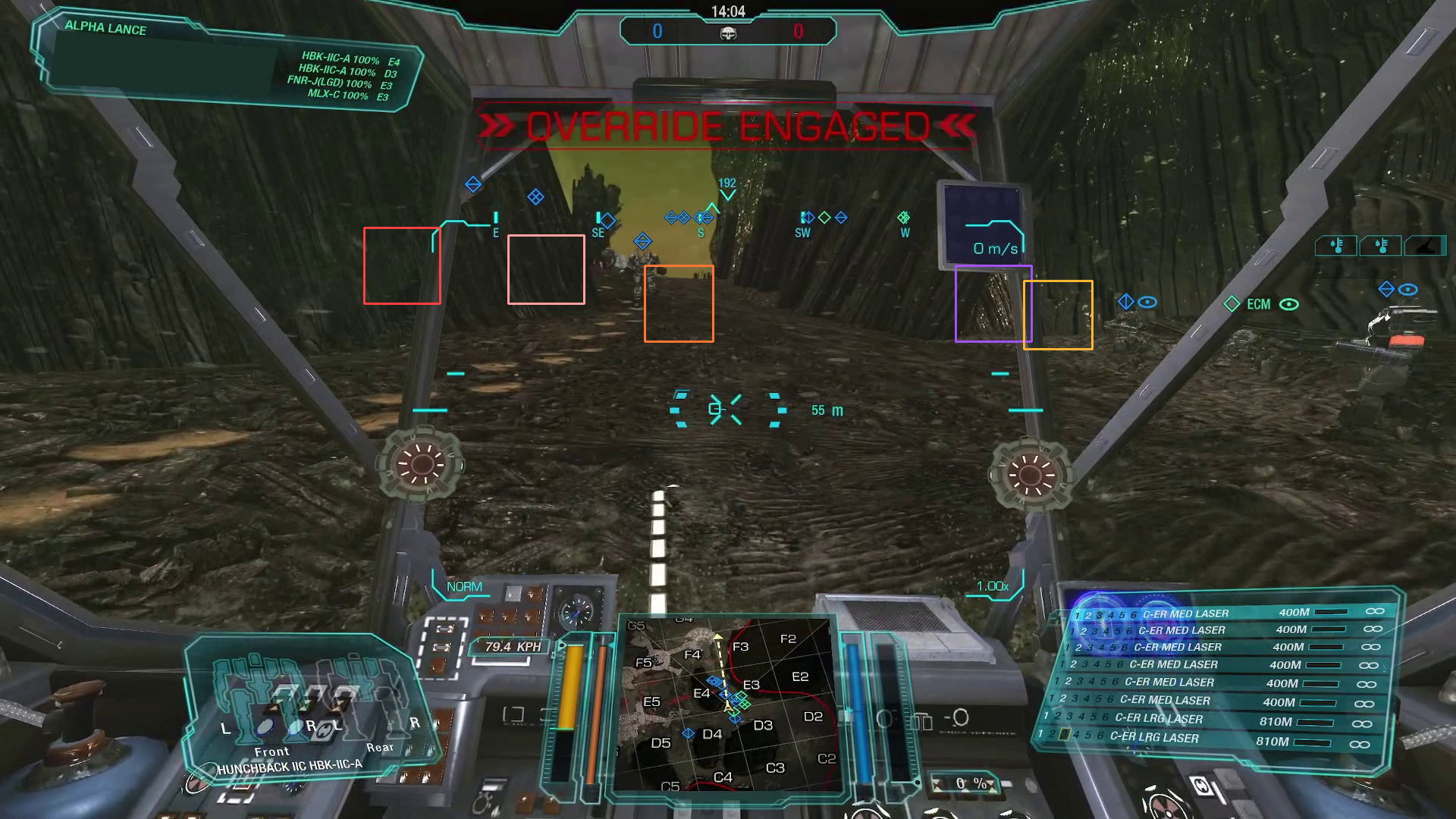
Summary
We have undergone quite a journey in this post. What then, are its takeaways?
The primary one, perhaps: for specialized use cases, such as, apparently, ours, there is still no free lunch. Most popular vision models are trained principally on "real world" images, with "real world" objects. No wonder – most of the use cases need those. For others, however, non-trivial work needs to be done, at least in the form of fine-tuning. Bummer on the one hand, yet on the other, a confirmation that a lot of interesting problem-solving is still there to explore.
The second takeaway flows from the first: even the models of the "large" corps, trained while burning through the equivalent electricity consumption of a small country (not an exaggeration), are unable to give answers to our problems for "free" – not because they are not deficient or borderline sci-fi at times, but they simply weren’t trained for this. And speaking of "free", were they even capable of performing the required tasks flawlessly, they’d still be prohibitively expensive for such a hobby use case – we’re talking dollars on the thousands of images/frames.
Finally, and most importantly, some conclusions relevant to the "offline" models we’ve gone through:
-
YOLO-World seemed to be the weakest for our use case, but even it can probably be made to work well with some fine-tuning, and not only for finding designators, but for mechs themselves. The latter, of course, presents a chicken-and-egg problem, as we need the data somehow.
-
Distilled VLMs such as Moondream work even better, and, perhaps in the near future, will be good enough to employ for the use case of detecting video-game-specific objects without any fine-tuning. We must, however, be mindful of their complexity and resource requirements, barely runnable on the majority of consumer-grade hardware. We cannot then unequivocally settle on them just yet.
-
Modern generalist segmentation models such as SAM 2 offer the most intriguing opportunity – sure, they’re not as convenient as auto-detecting target designators, but:
-
they potentially output more precise annotation information via the masks;
-
with a couple of days of work, the input data yield can surpass the autodetection approach, as we can locate and annotate arbitrary objects on the screen, not just the ones surrounded by a specific UI element;
-
however, we will need to wait for less power-hungry derivatives for this to work in an acceptable timeframe.
-
Overall, we’re still not quite in the singularity that’s being heralded as coming soon for some time now, not even for "simple" tasks such as computer vision. But that’s good! It means there’s still a lot of interesting work to be done, and a lot of interesting problems to solve.
In the next entries, we will proceed with that problem-solving. We’ll be using several approaches to precisely extract the target designators, and we will start doing what we should have been doing already – comparing the performance and resource usage of various approaches in a diligent manner. We probably will even revisit some of the models (or their derivatives) discussed here. For now – until next time!
def
column that can be wrangled with some basic NLP, but that is unlikely to bring any novel or contradicting conclusions.
Twitter
Google+
Facebook
Reddit
LinkedIn
StumbleUpon
Email